Rahimi Research Lab
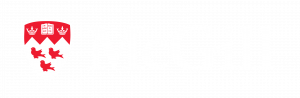
Inventory of AI Interventions in Community Based Primary Health Care
Paper 1
Paper Title: Type 2 diabetes screening test by means of a pulse oximeter
Authors or developers | Moreno, E. Lujan, M. J. Anyo Lujan, M. Torrres Rusinol, M. Juarez Fernandez, P. Nunez Manrique, P. Aragon Trivino, C. Miquel, M. Rodriguez, M. Gonzalez Burguillos, M. J. | |||||||||||||||
---|---|---|---|---|---|---|---|---|---|---|---|---|---|---|---|---|
Year of Publication | 2016 | |||||||||||||||
Full reference of the study | Moreno, Enrique Monte, et al. “Type 2 diabetes screening test by means of a pulse oximeter.” IEEE Transactions on Biomedical Engineering 64.2 (2016): 341-351. | |||||||||||||||
Abstract | In this paper, we propose a method for screening for the presence of type 2 diabetes by means of the signal obtained from a pulse oximeter. The screening system consists of two parts; the first analyses the signal obtained from the pulse oximeter, and the second consists of a machine-learning module. The system consists of a front end that extracts a set of features form the pulse oximeter signal. These features are based on physiological considerations. The set of features were the input of a machine-learning algorithm that determined the class of the input sample, i.e. whether the subject had diabetes or not. The machine-learning algorithms were random forests, gradient boosting, and linear discriminant analysis as benchmark. The system was tested on a database of 1; 157 subjects (two samples per subject) collected from five community health centres. The mean receiver operating characteristic (ROC) area found was 69:4% (median value 71:9% and range [75:4%?61:1%]), with a specificity=64% for a threshold that gave a sensitivity=65%. We present a screening method for detecting diabetes that has a performance comparable to the glycated haemoglobin (haemoglobin A1c HbA1c) test, does not require blood extraction, and yields results in less than five minutes. | |||||||||||||||
Country of Research | Spain | |||||||||||||||
Design of Study | Screening trial | |||||||||||||||
Duration of Study | Not specified,(spring of 2013) | |||||||||||||||
Name of Condition | Type 2 Diabetes | |||||||||||||||
Artificial Intelligence Technique Used | Random forest, fradient boosting | |||||||||||||||
Provider’s involvement in | Developing | |||||||||||||||
Accuracy of the AI Intervention | Not specified | |||||||||||||||
Patient-related Outcomes Assessed | Screening method for detecting diabetes that has a performance comparable to the glycated haemoglobin (haemoglobin A1c HbA1c) test, does not require blood extraction, and yields results in less than 5 min | |||||||||||||||
Primary Healthcare Worker Related Outcomes Assessed | Not specified | |||||||||||||||
Healthcare System-related Outcomes Assessed | Not specified | |||||||||||||||
Reached Target Population? | Yes | |||||||||||||||
Adoption | Not specified | |||||||||||||||
Implementation | Not specified | |||||||||||||||
Maintenance | No | |||||||||||||||
Key Conclusions | The work have presented a method of screening and diagnosis of diabetes based on the signal obtained from a photoplethysmogram PPG. One of the advantages of this method is that it is a fast test, the results can be obtained in 2 or 3 min, and the price is low because it needs only a PPG sensor and the computation can be done using either a low-cost computer or a smart phone. The procedure consists of obtaining a sample of the pulse oximeter signal of one minute in duration, which does not require of qualified personnel, and the whole process (including the computation of the results) takes less than five minutes (including possible repetitions of the measure). This is in contrast to plasma glucose measurements or glycated haemoglobin tests, which require the extraction of a blood sample, and laboratory measurements. | |||||||||||||||
Risk of Bias |
|
Paper 2
Paper Name: ProPath - A guideline based software for the implementation into the medical environment
Authors or developers | S. Klausner K. Entacher S. Kranzer A. Sönnichsen M. Flamm G. Fritsch | |||||||||||||||
---|---|---|---|---|---|---|---|---|---|---|---|---|---|---|---|---|
Year of Publication | 2014 | |||||||||||||||
Full reference of the study | S. Klausner, K. Entacher, S. Kranzer, A. Snnichsen, M. Flamm and G. Fritsch, “ProPath – A guideline based software for the implementation into the medical environment,” 2014 IEEE Canada International Humanitarian Technology Conference – (IHTC), Montreal, QC, 2014, pp. 1-6, doi: 10.1109/IHTC.2014.7147551. | |||||||||||||||
Abstract | Over the last decades, the amount of medical information has been growing rapidly. Online platforms such as patient’s and doctor’s blogs and forums and medical databases are widely and easily accessible to medical professionals as well as to the public. However, researching, filtering and evaluating the quality of this often overwhelming amount of data remains a challenge. Moreover, existing guidelines in the medical context are extensive and hardly applicable in the clinical context since reading and translation into clinical practice is time consuming [1][2]. Due to growing critical awareness among patients towards their medical treatment, there is an increased demand from internists, general practitioners, and other specialists, to explain medical conditions, treatment options and procedures in a more comprehensive fashion. In addition this discussion should be supported by the current state of clinical research. Expert systems could provide valuable support to fulfill these needs. Initial prototypes of expert systems in the inpatient arena were already implemented in the 1960’s in the context of clinical trials [3]. The main goal of these systems was to improve medical care by assisting in the medical decision process. However, most of these systems did not remain in clinical practice for a prolonged period of time. In most cases, the user interface of the software was too complex for daily use. Appropriate application and a detailed insight into these systems requires a lot of handbook knowledge. Therefore the initial hurdles for the integration of software into specific clinical application, faced by the potential users were too cumbersome. The main purpose of the project ProPath was to eliminate these issues and at the same time provide optimal clinical practice for the health care system in a variety of medical topics. Both in the outpatient and inpatient scenario, there is an increasing demand to support communication and to improve the distribution of – ublished knowledge and the application of practical experiences within the medical field. The main challenge to achieve that objective is to design an intuitive, user friendly software product that can be integrated into the current standard network environments. An example of successful implementation of a medical information system into clinical practice is the PROP system [4]. It is a medical decision support system, which has been designed, developed and implemented in Austria in the course of Reformpoolprojekt, in order to optimize the preoperative process. Since 2008, it is applied by general practicioners, pediatricians, clinicians and internists, in the state of Salzburg and was externally evaluated by the Paracelsus Medical University (PMU) in Salzburg. This paper provides an overview on how acquired knowledge can be utilized to reduce the complexity of designing and implementing clinical pathways (ProPath), supported by medical information or expert systems. Finally, statistical results evaluating PROP user-behavior are described. | |||||||||||||||
Country of Research | Austria | |||||||||||||||
Design of Study | Unclear (Not mentioned) | |||||||||||||||
Duration of Study | 2 years | |||||||||||||||
Name of Condition | Not applicable | |||||||||||||||
Artificial Intelligence Technique Used | Expert system (ProPath) | |||||||||||||||
Provider’s involvement in | Developing : Not specified,Testing : Not specified,Validating : Not specified | |||||||||||||||
Accuracy of the AI Intervention | Not specified | |||||||||||||||
Patient-related Outcomes Assessed | Not specified | |||||||||||||||
Primary Healthcare Worker Related Outcomes Assessed | Not specified | |||||||||||||||
Healthcare System-related Outcomes Assessed | Integration of Information and Communication Technology (lCT) Systems into a common network environment can improve medical care | |||||||||||||||
Reached Target Population? | Yes | |||||||||||||||
Adoption | Yes (number of providers i.e. PHC participating) : 14986 visits in outpatient department | |||||||||||||||
Implementation | Not specified | |||||||||||||||
Maintenance | Yes | |||||||||||||||
Key Conclusions | The expert system provides increased communication vetween inpatient and outpatient medical professionals. Moreover, the system can translate medical information in a testing proposal for each individual patient. | |||||||||||||||
Risk of Bias |
|
Paper 3
Paper Name: Tackling Missing Data in Community Health Studies Using Additive LS-SVM Classifier
Authors or developers | G. Wang Z. Deng K. S. Choi | |||||||||||||||
---|---|---|---|---|---|---|---|---|---|---|---|---|---|---|---|---|
Year of Publication | 2018 | |||||||||||||||
Full reference of the study | Tackling missing data in community health studies using additive LS-SVM classifier G Wang, Z Deng, KS Choi – IEEE journal of biomedical and health informatics, 2016 | |||||||||||||||
Abstract | Missing data is a common issue in community health and epidemiological studies. Direct removal of samples with missing data can lead to reduced sample size and information bias, which deteriorates the significance of the results. While data imputation methods are available to deal with missing data, they are limited in performance and could introduce noises into the dataset. Instead of data imputation, a novel method based on additive least square support vector machine (LS-SVM) is proposed in this paper for predictive modeling when the input features of the model contain missing data. The method also determines simultaneously the influence of the features with missing values on the classification accuracy using the fast leave-one-out cross-validation strategy. The performance of the method is evaluated by applying it to predict the quality of life (QOL) of elderly people using health data collected in the community. The dataset involves demographics, socioeconomic status, health history, and the outcomes of health assessments of 444 community-dwelling elderly people, with 5% to 60% of data missing in some of the input features. The QOL is measured using a standard questionnaire of the World Health Organization. Results show that the proposed method outperforms four conventional methods for handling missing data-case deletion, feature deletion, mean imputation, and K-nearest neighbor imputation, with the average QOL prediction accuracy reaching 0.7418. It is potentially a promising technique for tackling missing data in community health research and other applications. | |||||||||||||||
Country of Research | China | |||||||||||||||
Design of Study | Unclear | |||||||||||||||
Duration of Study | 1 year | |||||||||||||||
Name of Condition | Not specified,(Addressing missing data) | |||||||||||||||
Artificial Intelligence Technique Used | LS-SVM: Square support vector machine,(1. LS-SVM classifier 2. Case deletion 3. Feature deletion 4. Mean imputation 5. KNN imputation) | |||||||||||||||
Provider’s involvement in | Developing : e least square support vector machine classifier was developed to tackle the issues of missing data that are common in community health research | |||||||||||||||
Accuracy of the AI Intervention | Accuracy mean(S.D) 1. LS-SVM classifier:0.7430.021) 2. Case deletion: 0.7140.04 3. Feature deletion: 0.718 (0.040) 4. Mean imputation: 0.689 (0.016) 5. KNN imputation: 0.705 (0.029) | |||||||||||||||
Patient-related Outcomes Assessed | World Health Organization Questionnaire on Quality of Life: Short Form�Hong Kong version | |||||||||||||||
Primary Healthcare Worker Related Outcomes Assessed | Not specified | |||||||||||||||
Healthcare System-related Outcomes Assessed | Not specified | |||||||||||||||
Reached Target Population? | Not specified | |||||||||||||||
Adoption | Yes (number of providers i.e. PHC participating) : Data collected from a nurse-led mobile health center that provides primary and preventive healthcare services in the community | |||||||||||||||
Implementation | Additive LS-SVM classifier was developed to tackle the issues of missing data that are common in community health research. The handling of missing data and the construction of pattern classification model were carried out at the same time. | |||||||||||||||
Maintenance | Not specified (Unclear) | |||||||||||||||
Key Conclusions | A novel method based on additive least square support vector machine is proposed in this paper for predictive modeling when the input features of the model contain missing data. The method also determines simultaneously the influence of the features with missing values on the classification accuracy using the fast leave-one-out cross-validation strategy. The performance of the method is evaluated by applying it to predict the quality of life (QOL) of elderly people using health data collected in the community. The dataset involves demographics, socioeconomic status, health history, and the outcomes of health assessments of 444 community-dwelling elderly people, with 5% to 60% of data missing in some of the input features. The QOL is measured using a standard questionnaire of the World Health Organization. Results show that the proposed method outperforms four conventional methods for handling missing data�case deletion, feature deletion, mean imputation, and K-nearest neighbor imputation, with the average QOL prediction accuracy reaching 0.7418. It is potentially a promising technique for tackling missing data in community health research and other applications. | |||||||||||||||
Risk of Bias |
|
Paper 4
Paper Name: Rapid identification of familial hypercholesterolemia from electronic health records: the SEARCH study
Authors or developers | Safarova, Ms Liu, H Kullo, Ij | |||||||||||||||
---|---|---|---|---|---|---|---|---|---|---|---|---|---|---|---|---|
Year of Publication | 2016 | |||||||||||||||
Full reference of the study | Safarova, Maya S., Hongfang Liu, and Iftikhar J. Kullo. “Rapid identification of familial hypercholesterolemia from electronic health records: the SEARCH study.” Journal of clinical lipidology 10.5 (2016): 1230-1239. | |||||||||||||||
Abstract | Background: Little is known about prevalence, awareness, and control of familial hypercholesterolemia (FH) in the United States. Objective: To address these knowledge gaps, we developed an ePhenotyping algorithm for rapid identification of FH in electronic health records (EHRs) and deployed it in the Screening Employees And Residents in the Community for Hypercholesterolemia (SEARCH) study. Methods: We queried a database of 131,000 individuals seen between 1993 and 2014 in primary care practice to identify 5992 (mean age 52 +/- 13 years, 42% men) patients with low-density lipoprotein cholesterol (LDL-C) >190 mg/dL, triglycerides <400 mg/dL and without secondary causes of hyperlipidemia. Results: Our EHR-based algorithm ascertained the Dutch Lipid Clinic Network criteria for FH using structured data sets and natural language processing for family history and presence of FH stigmata on physical examination. Blinded expert review revealed positive and negative predictive values for the SEARCH algorithm at 94% and 97%, respectively. The algorithm identified 32 definite and 391 probable cases with an overall FH prevalence of 0.32% (1:310). Only 55% of the FH cases had a diagnosis code relevant to FH. Mean LDL-C at the time of FH ascertainment was 237 mg/dL; at follow-up, 70% (298 of 423) of patients were on lipid-lowering treatment with 80% achieving an LDL-C <100 mg/dL. Of treated FH patients with premature CHD, only 22% (48 of 221) achieved an LDL-C <70 mg/dL. Conclusions: In a primary care setting, we found the prevalence of FH to be 1:310 with low awareness and control. Further studies are needed to assess whether automated detection of FH in EHR improves patient outcomes. Copyright � 2016 National Lipid Association. | |||||||||||||||
Country of Research | USA | |||||||||||||||
Design of Study | Cohort study | |||||||||||||||
Duration of Study | 21 years,(June 1993-Dec 2014) | |||||||||||||||
Name of Condition | Familial hypercholesterolemia,(Additional history provided regarding familial history, secondary causes, personal history) | |||||||||||||||
Artificial Intelligence Technique Used | Mayo SEARCH algorithm (Natural language processing),(Algorithm mined lipid and non lipid criteria for familial hypercholesterolemia.) | |||||||||||||||
Provider’s involvement in | Developing : Not specified,Testing : Not specified,Validating : Not specified | |||||||||||||||
Accuracy of the AI Intervention | Sensitivity: 97% Specificity: 94% Positive predictive value: 94% Negative predictive value: 97% | |||||||||||||||
Patient-related Outcomes Assessed | Dutch Lipid Clinic Networkscoring system score,(Definite familial hypercholesterolemia: 32 (Dutch Lipid Clinic Networkscoring system score : 10.2 S.D: 1.7) Probable familial hypercholesterolemia: 391 (Dutch Lipid Clinic Networkscoring system score : 6.1 S.D: 0.4)) | |||||||||||||||
Primary Healthcare Worker Related Outcomes Assessed | Not specified | |||||||||||||||
Healthcare System-related Outcomes Assessed | Not specified | |||||||||||||||
Reached Target Population? | Yes | |||||||||||||||
Adoption | Yes (number of providers i.e. PHC participating) : Mayo Employee and Community Health system that delivers primary care to residents of Olmsted County and southeastern Minnesota | |||||||||||||||
Implementation | Not specified | |||||||||||||||
Maintenance | Not specified (Unclear) | |||||||||||||||
Key Conclusions | The study reveals that a natural language processing algorithm (Mayo SEARCH) had an high accuracy in ascertaining familial hypercholesterolemia cases among individuals with severe hypercholesterolemia. | |||||||||||||||
Risk of Bias |
|
Paper 5
Paper Name: A bioinformatics approach to identify patients with symptomatic peanut allergy using peptide microarray immunoassay
Authors or developers | Lin, J Bruni, Fm Fu, Z Maloney, J Bardina, L Boner, Al Gimenez, G Sampson, Ha | |||||||||||||||
---|---|---|---|---|---|---|---|---|---|---|---|---|---|---|---|---|
Year of Publication | 2012 | |||||||||||||||
Full reference of the study | Lin, Jing, et al. “A bioinformatics approach to identify patients with symptomatic peanut allergy using peptide microarray immunoassay.” Journal of allergy and clinical immunology 129.5 (2012): 1321-1328. | |||||||||||||||
Abstract | Background: Peanut allergy is relatively common, typically permanent, and often severe. Double-blind, placebo-controlled food challenge is considered the gold standard for the diagnosis of food allergy-related disorders. However, the complexity and potential of double-blind, placebo-controlled food challenge to cause life-threatening allergic reactions affects its clinical application. A laboratory test that could accurately diagnose symptomatic peanut allergy would greatly facilitate clinical practice. Objective: We sought to develop an allergy diagnostic method that could correctly predict symptomatic peanut allergy by using peptide microarray immunoassays and bioinformatic methods. Methods: Microarray immunoassays were performed by using the sera from 62 patients (31 with symptomatic peanut allergy and 31 who had outgrown their peanut allergy or were sensitized but were clinically tolerant to peanut). Specific IgE and IgG4 binding to 419 overlapping peptides (15 mers, 3 offset) covering the amino acid sequences of Ara h 1, Ara h 2, and Ara h 3 were measured by using a peptide microarray immunoassay. Bioinformatic methods were applied for data analysis. Results: Individuals with peanut allergy showed significantly greater IgE binding and broader epitope diversity than did peanut-tolerant individuals. No significant difference in IgG4 binding was found between groups. By using machine learning methods, 4 peptide biomarkers were identified and prediction models that can predict the outcome of double-blind, placebo-controlled food challenges with high accuracy were developed by using a combination of the biomarkers. Conclusions: In this study, we developed a novel diagnostic approach that can predict peanut allergy with high accuracy by combining the results of a peptide microarray immunoassay and bioinformatic methods. Further studies are needed to validate the efficacy of this assay in clinical practice. 2012 American Academy of Allergy, Asthma & Immunology. | |||||||||||||||
Country of Research | USA | |||||||||||||||
Design of Study | Screening trial | |||||||||||||||
Duration of Study | 6 years, 2001-2007 | |||||||||||||||
Name of Condition | Peanut allergy | |||||||||||||||
Artificial Intelligence Technique Used | Supervised machine learning models: Decesion tree, support vector machine (develop/train the prediction models and select a combination of the least number of peptides with the highest accuracy in classifying patients), For decision trees, the tree was pruned to 4 nodes to reach the best generalization performance. For support vector machines, the Gaussian kernel was selected, SD of the Gaussian kernel was set to 3, and Cbound was set to 10. Both classifiers were used for feature selection and subjected to 5-fold cross-validation as explained below. | |||||||||||||||
Providers’ involvement in | Developing : Not specified,Testing : Not specified,Validating : Not specified | |||||||||||||||
Accuracy of the AI Intervention | Specificity: 94%, Sensitivity: 87%, Accuracy: 90% | |||||||||||||||
Patient-related Outcomes Assessed | Serum sIgE levels to peanut allergens Ara h 1, Ara h 2, and Ara h 3 were determined by using ISAC, According to the ISAC data reported in the study both Ara h 1 and Ara h 3 were bound by approximately 13% of peanut-allergic patients and approximately 9% of peanut-tolerant patients and had very low or no classification power. Ara h 2 were bound by 20 peanut-allergic patients and only 1 peanut-tolerant patient. It had the highest classification power among Ara h 1, Ara h2, and Ara h 3, and it reached 74% sensitivity and 96% specificity in distinguishing peanut-allergic groups from peanut-tolerant groups. | |||||||||||||||
Primary Healthcare Worker Related Outcomes Assessed | Not specified | |||||||||||||||
Healthcare System-related Outcomes Assessed | Not specified | |||||||||||||||
Reached Target Population? | Yes | |||||||||||||||
Adoption | Yes (number of providers i.e. PHC participating) : Data gathered from a primary care centre | |||||||||||||||
Implementation | Not specified | |||||||||||||||
Maintenance | Not specified | |||||||||||||||
Key Conclusions | A novel peanut allergy diagnostic approach with higher accuracy than current allergy tests was developed by employing peptide microarray immunoassays and bioinformatics. This method may be useful for clinical allergy testing in the future | |||||||||||||||
Risk of Bias |
|
Paper 6
Paper Name: Medicine in words and numbers: a cross-sectional survey comparing probability assessment scales
Authors or developers | Witteman, Cl Renooij, S Koele, P | |||||||||||||||
---|---|---|---|---|---|---|---|---|---|---|---|---|---|---|---|---|
Year of Publication | 2007 | |||||||||||||||
Full reference of the study | Witteman, C. L., Renooij, S., & Koele, P. (2007). Medicine in words and numbers: a cross-sectional survey comparing probability assessment scales. BMC Medical Informatics and Decision Making, 7(1), 13. | |||||||||||||||
Abstract | BACKGROUND: In the complex domain of medical decision making, reasoning under uncertainty can benefit from supporting tools. Automated decision support tools often build upon mathematical models, such as Bayesian networks. These networks require probabilities which often have to be assessed by experts in the domain of application. Probability response scales can be used to support the assessment process. We compare assessments obtained with different types of response scale. METHODS: General practitioners (GPs) gave assessments on and preferences for three different probability response scales: a numerical scale, a scale with only verbal labels, and a combined verbal-numerical scale we had designed ourselves. Standard analyses of variance were performed. RESULTS: No differences in assessments over the three response scales were found. Preferences for type of scale differed: the less experienced GPs preferred the verbal scale, the most experienced preferred the numerical scale, with the groups in between having a preference for the combined verbal-numerical scale. CONCLUSION: We conclude that all three response scales are equally suitable for supporting probability assessment. The combined verbal-numerical scale is a good choice for aiding the process, since it offers numerical labels to those who prefer numbers and verbal labels to those who prefer words, and accommodates both more and less experienced professionals. | |||||||||||||||
Country of Research | Netherlands | |||||||||||||||
Design of Study | Screening trial | |||||||||||||||
Duration of Study | Not specified | |||||||||||||||
Name of Condition | Not applicable | |||||||||||||||
Artificial Intelligence Technique Used | Real life Bayesian network | |||||||||||||||
Provider’s involvement in | Developing : Not specified,Testing : GP,Validating : GP | |||||||||||||||
Accuracy of the AI Intervention | Not tested | |||||||||||||||
Patient-related Outcomes Assessed | Not specified | |||||||||||||||
Primary Healthcare Worker Related Outcomes Assessed | Diagnostic, prognostic or therapeutic alternatives judged on the basis of probability questions (verbal, numerical, verbal-numerical),A vignette represented a medical situation followed by three probabilistic choices that the GP could make i.e. what was the probability of the patient suffering from this disease?. Moreover, the preference regarding verbal, numerical, numerical-verbal scale was also inquired. | |||||||||||||||
Healthcare System-related Outcomes Assessed | Not specified | |||||||||||||||
Reached Target Population? | Yes : Not specified | |||||||||||||||
Adoption | Yes (number of providers i.e. PHC participating) : Not specified | |||||||||||||||
Implementation | The study acquired data for developing automated decision making model. Therefore, not implemented. | |||||||||||||||
Maintenance | Not specified (Unclear) | |||||||||||||||
Key Conclusions | The study reported that all 3 probability response scales (numerical ,verbal label, combined numerical-verbal scale) supported probability assessment. However, the combined verbal-numerical scale would be preferred to develop automatic decesion support tool with Bayesian network as it accomodates to the need of both more experienced and less experienced GP’s in primary care. | |||||||||||||||
Risk of Bias |
|
Paper 7
Paper Name: Development of an Automatic Diagnostic Algorithm for Pediatric Otitis Media
Authors or developers | Tran, T. T. Fang, T. Y. Pham, V. T. Lin, C. Wang, P. C. Lo, M. T. | |||||||||||||||
---|---|---|---|---|---|---|---|---|---|---|---|---|---|---|---|---|
Year of Publication | 2018 | |||||||||||||||
Full reference of the study | Tran, Thi-Thao, et al. “Development of an automatic diagnostic algorithm for pediatric otitis media.” Otology & Neurotology 39.8 (2018): 1060-1065. | |||||||||||||||
Abstract | HYPOTHESIS: The artificial intelligence and image processing technology can develop automatic diagnostic algorithm for pediatric otitis media (OM) with accuracy comparable to that from well-trained otologists. BACKGROUND: OM is a public health issue that occurs commonly in pediatric population. Caring for OM may incur significant indirect cost that stems mainly from loss of school or working days seeking for medical consultation. It makes great sense for the homecare of OM. In this study, we aim to develop an automatic diagnostic algorithm for pediatric OM. METHODS: A total of 1,230 otoscopic images were collected. Among them, 214 images diagnosed of acute otitis media (AOM) and otitis media with effusion (OME) are used as the database for image classification in this study. For the OM image classification system, the image database is randomly partitioned into the test and train subsets. Of each image in the train and test sets, the desired eardrum image region is first segmented, then multiple image features such as color, and shape are extracted. The multitask joint sparse representation-based classification to combine different features of the OM image is used for classification. RESULTS: The multitask joint sparse representation algorithm was applied for the classification of the AOM and OME images. The approach is able to differentiate the OME from AOM images and achieves the classification accuracy as high as 91.41%. CONCLUSION: Our results demonstrated that this automatic diagnosis algorithm has acceptable accuracy to diagnose pediatric OM. The cost-effective algorithm can assist parents for early detection and continuous monitoring at home to decrease consequence of the disease. | |||||||||||||||
Country of Research | Taiwan | |||||||||||||||
Design of Study | Screening trial | |||||||||||||||
Duration of Study | Not specified | |||||||||||||||
Name of Condition | Not specified | |||||||||||||||
Artificial Intelligence Technique Used | machine learning-based image classification approaches | |||||||||||||||
Providers’ involvement in | Developing | |||||||||||||||
Accuracy of the AI Intervention | 91.41%, Classification accuracy as high as 91.41%. | |||||||||||||||
Patient-related Outcomes Assessed | Automatic diagnosis algorithm has acceptable accuracy to diagnose pediatric otitis media. The cost-effective algorithm can assist parents for early detection and continuous monitoring at home to decrease consequence of the disease | |||||||||||||||
Primary Healthcare Worker Related Outcomes Assessed | Not specified | |||||||||||||||
Healthcare System-related Outcomes Assessed | Diagnosis algorithm can be installed in a portable device to assist parents with initial detection and monitor the disease progress to reduce burden and expenses for medical consultation | |||||||||||||||
Reached Target Population? | Yes | |||||||||||||||
Adoption | Not mentioned/td> | |||||||||||||||
Implementation | Yes | |||||||||||||||
Maintenance | No | |||||||||||||||
Key Conclusions | Automatic diagnosis algorithm has acceptable accuracy to diagnose pediatric otitis media. The cost-effective algorithm can assist parents for early detection and continuous monitoring at home to decrease consequence of the disease. | |||||||||||||||
Risk of Bias |
|
Paper 8
Paper Name: Using natural language processing for identification of herpes zoster ophthalmicus cases to support population-based study
Authors or developers | Zheng, C. Luo, Y. Mercado, C. Sy, L. Jacobsen, S. J. Ackerson, B. Lewin, B. Tseng, H. F. | |||||||||||||||
---|---|---|---|---|---|---|---|---|---|---|---|---|---|---|---|---|
Year of Publication | 2018 | |||||||||||||||
Full reference of the study | Zheng, Chengyi, et al. “Using natural language processing for identification of herpes zoster ophthalmicus cases to support population‐based study.” Clinical & experimental ophthalmology 47.1 (2019): 7-14. | |||||||||||||||
Abstract | IMPORTANCE: Diagnosis codes are inadequate for accurately identifying herpes zoster (HZ) ophthalmicus (HZO). There is significant lack of population-based studies on HZO due to the high expense of manual review of medical records. BACKGROUND: To assess whether HZO can be identified from the clinical notes using natural language processing (NLP). To investigate the epidemiology of HZO among HZ population based on the developed approach. DESIGN: A retrospective cohort analysis. PARTICIPANTS: A total of 49914 southern California residents aged over 18years, who had a new diagnosis of HZ. METHODS: An NLP-based algorithm was developed and validated with the manually curated validation data set (n =461). The algorithm was applied on over 1 million clinical notes associated with the study population. HZO versus non-HZO cases were compared by age, sex, race and co-morbidities. MAIN OUTCOME MEASURES: We measured the accuracy of NLP algorithm. RESULTS: NLP algorithm achieved 95.6% sensitivity and 99.3% specificity. Compared to the diagnosis codes, NLP identified significant more HZO cases among HZ population (13.9% vs. 1.7%). Compared to the non-HZO group, the HZO group was older, had more males, had more Whites and had more outpatient visits. CONCLUSIONS AND RELEVANCE: We developed and validated an automatic method to identify HZO cases with high accuracy. As one of the largest studies on HZO, our finding emphasizes the importance of preventing HZ in the elderly population. This method can be a valuable tool to support population-based studies and clinical care of HZO in the era of big data. | |||||||||||||||
Country of Research | USA | |||||||||||||||
Design of Study | Cohort study,Unclear,Retrospective | |||||||||||||||
Duration of Study | 2 years 11 months (1 January 2012 and 31 December 2014) | |||||||||||||||
Name of Condition | Herpes zoster ophthalmicus (Additional morbidities also mentioned including asthma, allergy) | |||||||||||||||
Artificial Intelligence Technique Used | Natural language processing (The machine learning modules included sentence splitting, tokenization, part-of-speech tagging, parsing and indexing) | |||||||||||||||
Provider’s involvement in | Developing : Not specified,Testing : Not specified,Validating : Not specified | |||||||||||||||
Accuracy of the AI Intervention | Sensitivity: 95.6%, Specificity, 99.3%, positive predictive value: 93.5%, negative predictive value: 99.5%, positive likelihood ratio: 132.5, negative likelihood ratio: 0.04 | |||||||||||||||
Patient-related Outcomes Assessed | Prevalance herpes: 1.7% (Hispanics, Blacks and people of mixed race were less likely to have herpes zoster opthalmicus compared to whites) | |||||||||||||||
Primary Healthcare Worker Related Outcomes Assessed | Not specified | |||||||||||||||
Healthcare System-related Outcomes Assessed | Not specified | |||||||||||||||
Reached Target Population? | Yes | |||||||||||||||
Adoption | Not specified | |||||||||||||||
Implementation | Not implemented | |||||||||||||||
Maintenance | Not specified (Unclear) | |||||||||||||||
Key Conclusions | A natural language processing algorithm was tested and validated to diagnose herpes zoster opthalmicus with high accuracy. | |||||||||||||||
Risk of Bias |
|
Paper 9
Paper Name: A Machine Learning Recommender System to Tailor Preference Assessments to Enhance Person-Centered Care Among Nursing Home Residents
Authors or developers | Gannod, G. C. Abbott, K. M. Van Haitsma, K. Martindale, N. Heppner, A. | |||||||||||||||
---|---|---|---|---|---|---|---|---|---|---|---|---|---|---|---|---|
Year of Publication | 2018 | |||||||||||||||
Full reference of the study | Gannod, G. C., Abbott, K. M., Van Haitsma, K., Martindale, N., & Heppner, A. (2019). A Machine Learning Recommender System to Tailor Preference Assessments to Enhance Person-Centered Care Among Nursing Home Residents. The Gerontologist, 59(1), 167-176. | |||||||||||||||
Abstract | Background and Objectives: Nursing homes (NHs) using the Preferences for Everyday Living Inventory (PELI-NH) to assess important preferences and provide person-centered care find the number of items (72) to be a barrier to using the assessment. Research Design and Methods: Using a sample of n = 255 NH resident responses to the PELI-NH, we used the 16 preference items from the MDS 3.0 Section F to develop a machine learning recommender system to identify additional PELI-NH items that may be important to specific residents. Much like the Netflix recommender system, our system is based on the concept of collaborative filtering whereby insights and predictions (e.g., filters) are created using the interests and preferences of many users. The algorithm identifies multiple sets of “you might also like” patterns called association rules, based upon responses to the 16 MDS preferences that recommends an additional set of preferences with a high likelihood of being important to a specific resident. Results: In the evaluation of the combined apriori and logistic regression approach, we obtained a high recall performance (i.e., the ratio of correctly predicted preferences compared with all predicted preferences and nonpreferences) and high precision (i.e., the ratio of correctly predicted rules with respect to the rules predicted to be true) of 80.2% and 79.2%, respectively. Discussion and Implications: The recommender system successfully provides guidance on how to best tailor the preference items asked of residents and can support preference capture in busy clinical environments, contributing to the feasibility of delivering person-centered care. | |||||||||||||||
Country of Research | USA | |||||||||||||||
Design of Study | Unclear | |||||||||||||||
Duration of Study | Not specified | |||||||||||||||
Name of Condition | Not specified, Tailor made care approach to enhance person centered care | |||||||||||||||
Artificial Intelligence Technique Used | Combined Apriori algorithm and a logistic regression configured with a generalized linear regression model | |||||||||||||||
Providers’ involvement in | Developing : Not specified,Testing : Not specified,Validating : Not specified | |||||||||||||||
Accuracy of the AI Intervention | 70%, Recall = .8021, precision = .7919, accuracy = .6979, and F1 -score = .7953. | |||||||||||||||
Patient-related Outcomes Assessed | Not specified | |||||||||||||||
Primary Healthcare Worker Related Outcomes Assessed | Not specified | |||||||||||||||
Healthcare System-related Outcomes Assessed | Not specified | |||||||||||||||
Reached Target Population? | Yes | |||||||||||||||
Adoption | Yes (number of providers i.e. PHC participating) : 255 | |||||||||||||||
Implementation | Not specified | |||||||||||||||
Maintenance | Not specified | |||||||||||||||
Key Conclusions | The recommender system discussed in the study provides guidance on how to best tailor the preference items asked of residents and can support preference capture in busy clinical environments, contributing to the feasibility of delivering person-centered care | |||||||||||||||
Risk of Bias |
|
Paper 10
Paper Name: A web-based prediction score for head and neck cancer referrals
Authors or developers | Lau, K. Wilkinson, J. Moorthy, R. | |||||||||||||||
---|---|---|---|---|---|---|---|---|---|---|---|---|---|---|---|---|
Year of Publication | 2018 | |||||||||||||||
Full reference of the study | Lau, K., Wilkinson, J., & Moorthy, R. (2018). A web‐based prediction score for head and neck cancer referrals. Clinical Otolaryngology, 43(4), 1043-1049. | |||||||||||||||
Abstract | OBJECTIVE: Following the announcement of the NHS Cancer Plan in 2000, anyone suspected of having cancer has to be seen by a specialist within 2 weeks of referral. Since this introduction, studies have shown that only 6.3%-14.6% of 2-week referrals were diagnosed with a head and neck cancer and that majority of the cancer diagnoses were via other referral routes. These studies suggest that the referral scheme is not currently cost-effective. Our aim is to develop a scoring system that determines the risk of head and neck cancer in a patient, which can then be used to aid GP referrals. DESIGN: Retrospective data were collected from 1075 patients with 2-week head and neck cancer referrals from general practitioners. The retrospective data collected included patients’ demographics, risk factors and relevant investigations. The data were used as input into a logistic regression to arrive at our model. Our approach included data analysis, machine learning techniques, statistical inference and model validation metrics to arrive at the best performing model. The model was then tested with more data from 235 prospective patients. RESULTS: Using our results from the logistic regression, we created a web-based tool that GPs can use to calculate their patient’s probability of cancer and use this result to assist in their decision regarding referral. Our prototype can be seen in Figure 2. CONCLUSION: We have created a prototype scoring system that can be hosted online to assist GPs with their referrals with a sensitivity of 31% and specificity of 92%. While we acknowledge that there are several limitations to our model, we believe we have created a novel preliminary scoring system that has the potential to be improved dramatically with further data and be very helpful for GPs in a long run. | |||||||||||||||
Country of Research | United Kingdom | |||||||||||||||
Design of Study | Cohort study,Unclear : Retrospective cohort study | |||||||||||||||
Duration of Study | Birmingham site: 1 year, 01/07/2009–01/07/2010 Slogh site: 4 months, 1/4/2013–31/8/2013 | |||||||||||||||
Name of Condition | Head & neck cancer referral, Additionally subgroup distribution was made on the basis of following habits: smoking, alcohol consumption, hoarse voice, neck lump, dysphagia, weight loss, oral ulcer etc. | |||||||||||||||
Artificial Intelligence Technique Used | Logistic regression | |||||||||||||||
Providers’ involvement in | Developing : Not specified,Testing : General Physicians referral,Validating : Not specified | |||||||||||||||
Accuracy of the AI Intervention | Not specified, 16 patients referred during validation (True positive: 5 (31%), False positive: 11, False negative: 18 (78%)), Thereafter, revision of model was performed on the basis of symptom i.e. thyroid swelling. False negative during re-evaluation reduced to 70%) | |||||||||||||||
Patient-related Outcomes Assessed | True positive and false positive for cancer referraal | |||||||||||||||
Primary Healthcare Worker Related Outcomes Assessed | Not specified | |||||||||||||||
Healthcare System-related Outcomes Assessed | Not specified | |||||||||||||||
Reached Target Population? | Yes | |||||||||||||||
Adoption | Not specified : General physicians participated in the study from 2 different hospitals in UK (number not specified) | |||||||||||||||
Implementation | Not specified | |||||||||||||||
Maintenance | Yes : The authors mentioned that additional data is needed for further validating, modifying and advancing the web based referral platform. | |||||||||||||||
Key Conclusions | This study has described the process of developing a web-based referral tool that GPs can use when referring a patient to the head and neck 2-week wait clinic | |||||||||||||||
Risk of Bias |
|
Paper 11
Paper Name :Long-term outcomes of a large, prospective observational cohort of older adults with back pain
Authors or developers | Jarvik, J. G. Gold, L. S. Tan, K. Friedly, J. L. Nedeljkovic, S. S. Comstock, B. A. Deyo, R. A. Turner, J. A. Bresnahan, B. W. Rundell, S. D. James, K. T. Nerenz, D. R. Avins, A. L. Bauer, Z. Kessler, L. Heagerty, P. J. | |||||||||||||||
---|---|---|---|---|---|---|---|---|---|---|---|---|---|---|---|---|
Year of Publication | 2018 | |||||||||||||||
Full reference of the study | Jarvik, J. G., Gold, L. S., Tan, K., Friedly, J. L., Nedeljkovic, S. S., Comstock, B. A., … & James, K. T. (2018). Long-term outcomes of a large, prospective observational cohort of older adults with back pain. The Spine Journal, 18(9), 1540-1551. | |||||||||||||||
Abstract | BACKGROUND CONTEXT: Although back pain is common among older adults, there is relatively little research on the course of back pain in this age group. PURPOSE: Our primary goals were to report 2-year outcomes of older adults initiating primary care for back pain and to examine the relative importance of patient factors versus medical interventions in predicting 2-year disability and pain. STUDY DESIGN/SETTING: This study used a predictive model using data from a prospective, observational cohort from a primary care setting. PATIENT SAMPLE: The study included patients aged >=65 years at the time of new primary care visits for back pain. OUTCOME MEASURES: Self-reported 2-year disability (Roland-Morris Disability Questionnaire [RDQ]) and back pain (0-10 numerical rating scale [NRS]). METHODS: We developed our models using a machine learning least absolute shrinkage and selection operator approach. We evaluated the predictive value of baseline characteristics and the incremental value of interventions that occurred between 0 and 90 days, and the change in patient disability and pain from 0 to 90 days. Limitations included confounding by indication and unmeasured confounding. RESULTS: Of 4,665 patients (89%) with follow-up, both RDQ (from mean 9.6 [95% confidence interval {CI} 9.4-9.7] to mean 8.3 [95% CI 8.0-8.5]) and back pain NRS (from mean 5.0 [95% CI 4.9-5.1] to mean 3.5 [95% CI 3.4-3.6]) scores improved slightly. Only 16% (15%-18%) reported no back pain-related disability or back pain at 2 years after initial visits. Regression model parameters explained 40% of the variation (R2) in 2-year RDQ scores, and the addition of 0- to 3-month change in RDQ score and pain improved prediction (R2=51%). The most consistent predictors of 2-year RDQ scores and back pain NRS scores were 0- to 90-day change in each respective outcome and patient confidence in improvement. Patients experienced 50% and 43% improvement in back pain and disability, respectively, 2 years after their initial visit. However, fewer than 20% of patients had complete resolution of their back pain and disability at that time. CONCLUSIONS: Baseline patient factors were more important than early interventions in explaining disability and pain after 2 years. | |||||||||||||||
Country of Research | USA | |||||||||||||||
Design of Study | Cohort study,Observational study,Unclear, prospective | |||||||||||||||
Duration of Study | Not specified | |||||||||||||||
Name of Condition | Low back pain | |||||||||||||||
Artificial Intelligence Technique Used | Minimization of the Schwarz Bayesian Criterion used to select the final predictive model | |||||||||||||||
Provider’s involvement in | Developing : Not specified,Testing : Not specified,Validating : Not specified | |||||||||||||||
Accuracy of the AI Intervention | Prediction 2 year 30% Back pain improvement: AUC Model 1, 2: 0.66, AUC Model 2, 3: 0.69 Prediction of 2-year 30% RolandMorris disability improvement: Model 1: 0.67, Model 2: 0.69, Model 3: 0.76 | |||||||||||||||
Patient-related Outcomes Assessed | Pain-related characteristics: modified RolandMorris Disability Questionnaire, the average back pain intensity and average leg pain intensity in the past week on a 0�10 numerical rating scale, the Brief Pain Inventory (BPI) Activity Interference Scale. Psychological distress: the four-item PHQ-4 (0�12) measure of anxiety and depressive symptoms, Health-related quality of life (HRQoL): European Quality of Life 5 Dimension (EQ5D), including both the quality of life index (0�1) (European Quality of Life 5 Dimension Index [EQ5D Index]) and the visual analog scale (European Quality of Life 5 Dimension Visual Analog Scale [EQ5D VAS]), Falls: the number of falls in the past 3 weeks and how many resulted in injury, from the Behavioral Risk Factor Surveillance System (BRFSS) survey, Body mass index, Quan comorbidity score, baseline diagnosis, number of relative value units, spine related interventions, opioid prescriptions, days from index visit to consent, chronic pain risk score, back complaints in the elders trial, Of 4,665 patients (89%) with follow-up, both Roland morris disability questionnaire (from mean 9.6 [95% confidence interval {CI} 9.4�9.7] to mean 8.3 [95% CI 8.0�8.5]) and back pain numerical rating scale (from mean 5.0 [95% CI 4.9�5.1] to mean 3.5 [95% CI 3.4�3.6]) scores improved slightly. Only 16% (15%-18%) reported no back pain-related disability or back pain at 2 years after initial visits. | |||||||||||||||
Primary Healthcare Worker Related Outcomes Assessed | Not specified,Our primary outcome was 2-year RDQ score (continuous variable), and secondary outcomes were back pain intensity rating (continuous variable), dichotomous 30% RDQ improvement, and dichotomous 30% back pain intensity improvement. | |||||||||||||||
Healthcare System-related Outcomes Assessed | Not specified | |||||||||||||||
Reached Target Population? | Yes | |||||||||||||||
Adoption | Not specified , Data from primary care centers included (Harvard Vanguard (Boston), Henry Ford HealthSystem (Detroit), and Kaiser-Permanente Northern California) | |||||||||||||||
Implementation | Not specified | |||||||||||||||
Maintenance | No : Not specified | |||||||||||||||
Key Conclusions | The study explains that the baseline patient factors were more important than early interventions in explaining disability and pain after 2 year | |||||||||||||||
Risk of Bias |
|
Paper 12
Paper Name: Innovative Informatics Approaches for Peripheral Artery Disease: Current State and Provider Survey of Strategies for Improving Guideline-Based Care
Authors or developers | Chaudhry, A. P. Afzal, N. Abidian, M. M. Mallipeddi, V. P. Elayavilli, R. K. Scott, C. G. Kullo, I. J. Wennberg, P. W. Pankratz, J. J. Liu, H. Chaudhry, R. Arruda-Olson, A. M. | |||||||||||||||
---|---|---|---|---|---|---|---|---|---|---|---|---|---|---|---|---|
Year of Publication | 2018 | |||||||||||||||
Full reference of the study | Chaudhry, Alisha P., et al. “Innovative informatics approaches for peripheral artery disease: current state and provider survey of strategies for improving guideline-based care.” Mayo Clinic Proceedings: Innovations, Quality & Outcomes 2.2 (2018): 129-136. | |||||||||||||||
Abstract | Objective: To quantify compliance with guideline recommendations for secondary prevention in peripheral artery disease (PAD) using natural language processing (NLP) tools deployed to an electronic health record (EHR) and investigate provider opinions regarding clinical decision support (CDS) to promote improved implementation of these strategies. Patients and Methods: Natural language processing was used for automated identification of moderate to severe PAD cases from narrative clinical notes of an EHR of patients seen in consultation from May 13, 2015, to July 27, 2015. Guideline-recommended strategies assessed within 6 months of PAD diagnosis included therapy with statins, antiplatelet agents, angiotensin-converting enzyme inhibitors or angiotensin receptor blockers, and smoking abstention. Subsequently, a provider survey was used to assess provider knowledge regarding PAD clinical practice guidelines, comfort in recommending secondary prevention strategies, and potential role for CDS. Results: Among 73 moderate to severe PAD cases identified by NLP, only 12 (16%) were on 4 guideline-recommended strategies. A total of 207 of 760 (27%) providers responded to the survey; of these 141 (68%) were generalists and 66 (32%) were specialists. Although 183 providers (88%) managed patients with PAD, 51 (25%) indicated they were uncomfortable doing so; 138 providers (67%) favored the development of a CDS system tailored for their practice and 146 (71%) agreed that an automated EHR-derived mortality risk score calculator for patients with PAD would be helpful. Conclusion: Natural language processing tools can identify cases from EHRs to support quality metric studies. Findings of this pilot study demonstrate gaps in application of guideline-recommended strategies for secondary risk prevention for patients with moderate to severe PAD. Providers strongly support the development of CDS systems tailored to assist them in providing evidence-based care to patients with PAD at the point of care. | |||||||||||||||
Country of Research | USA | |||||||||||||||
Design of Study | Cohort study | |||||||||||||||
Duration of Study | 3 months, May 13, 2015, to July 27, 2015 | |||||||||||||||
Name of Condition | Peripheral Artery Disease, Diabetes, hypertension as aditional comorbidities mentioned | |||||||||||||||
Artificial Intelligence Technique Used | Natural language processing | |||||||||||||||
Providers’ involvement in | Developing : Not specified,Testing : Not specified,Validating : Not specified | |||||||||||||||
Accuracy of the AI Intervention | Not specified | |||||||||||||||
Patient-related Outcomes Assessed | Study demonstrate gaps in application of guideline-recommended strategies for secondary risk prevention for patients with moderate to severe peripheral artery disease | |||||||||||||||
Primary Healthcare Worker Related Outcomes Assessed | The provider survey had an overall response rate of 27% (207 of 760 providers). Among these 207 responders, 123 (59%) were staff physicians, 58 (28%) were nurse practitioners or physician assistants, and 26 (13%) residents or fellows., Within the responder group, 141 (68%) were generalists and 66 (32%) were specialists (25% cardiology, 5% vascular medicine, and 2% vascular surgery). A total of 183 (88%) respondents currently cared for patients with peripheral arterial disease, and 129 (62%) reported seeing an average of 1 to 5 patients with peripherial arterial disease per month. | |||||||||||||||
Healthcare System-related Outcomes Assessed | This pilot study demonstrate gaps in application of guideline-recommended strategies for secondary risk prevention for patients with moderate to severe peripheral artery disease. Providers strongly support the development of clinical decision support systems tailored to assist them in providing evidence-based care to patients with peripheral artery disease at the point of care. | |||||||||||||||
Reached Target Population? | Yes | |||||||||||||||
Adoption | Not specified | |||||||||||||||
Implementation | Yes | |||||||||||||||
Maintenance | Not specified | |||||||||||||||
Key Conclusions | The natural language processing tool used in the study identified cases from electronic health records for the application of guideline recommended strategies for secondary risk prevention for patients with peripheral arterial disease. Mroeover, provider survey reported a strong support for the development of such clinical decision support system. | |||||||||||||||
Risk of Bias |
|
Paper 13
Paper Name: A new computational intelligence approach to detect autistic features for autism screening
Authors or developers | Thabtah, F. Kamalov, F. Rajab, K. | |||||||||||||||
---|---|---|---|---|---|---|---|---|---|---|---|---|---|---|---|---|
Year of Publication | 2018 | |||||||||||||||
Abstract | Autism Spectrum Disorder (ASD) is one of the fastest growing developmental disability diagnosis. General practitioners (GPs) and family physicians are typically the first point of contact for patients or family members concerned with ASD traits observed in themselves or their family member. Unfortunately, some families and adult patients are unaware of ASD traits that may be exhibited and as a result do not seek out necessary diagnostic services or contact their GP. Therefore, providing a quick, accessible, and simple tool utilizing items related to ASD to these families may increase the likelihood they will seek professional assessment and is vital to the early detection and treatment of ASD. This study aims at identifying fewer, albeit influential, features in common ASD screening methods in order to achieve efficient screening as demands on evaluating the items’ influences on ASD within existing tools is urgent. To achieve this aim, a computational intelligence method called Variable Analysis (Va) is proposed that considers feature-to-class correlations and reduces feature-to-feature correlations. The results of the Va have been verified using two machine learning algorithms by deriving automated classification systems with respect to specificity, sensitivity, positive predictive values (PPVs), negative predictive values (NPVs), and predictive accuracy. Experimental results using cases and controls related to items in three common screening methods, along with features related to individuals, have been analysed and compared with results obtained from other common filtering methods. The results exhibited that Va was able to derive fewer numbers of features from adult, adolescent, and child screening methods yet maintained competitive predictive accuracy, sensitivity, and specificity rates. | |||||||||||||||
Full reference of the study | Thabtah, Fadi, Firuz Kamalov, and Khairan Rajab. “A new computational intelligence approach to detect autistic features for autism screening.” International journal of medical informatics 117 (2018): 112-124. | |||||||||||||||
Country of Research | University of Cambridge United Kingdom | |||||||||||||||
Design of Study | Unclear | |||||||||||||||
Duration of Study | Not specified | |||||||||||||||
Name of Condition | Autism spectrum disorder, 194 cases with family members diagnosed with ASD and 165 cases of individuals born with jaundice | |||||||||||||||
Artificial Intelligence Technique Used | Variable analysis, 5 filtration methods compared, Chi square, Information gain, correlation feature set, Correlation, all of them | |||||||||||||||
Providers’ involvement in | Developing : Not specified,Testing : Not specified,Validating : Not specified | |||||||||||||||
Accuracy of the AI Intervention | Adolscent: specificity: 87.3%, sensitivity: 80.95%, positive predictive values: 80.95%, negative predictive values: 84.13%, and postive predictive accuracy: 80% Adult: sensitivity: 80.95-82.54% (C4.5 and ripper algorithm) | |||||||||||||||
Patient-related Outcomes Assessed | Not specified | |||||||||||||||
Primary Healthcare Worker Related Outcomes Assessed | Not specified | |||||||||||||||
Healthcare System-related Outcomes Assessed | Not specified | |||||||||||||||
Reached Target Population? | Yes | |||||||||||||||
Adoption | Not specified : The tool was developed to be used in a primary care setting i.e. by general practitioners, patients, medical staff etc. | |||||||||||||||
Implementation | Not specified | |||||||||||||||
Maintenance | Not specified | |||||||||||||||
Key Conclusions | A self administered autism spectrum disorder assessment tool that could be used by patient, caregiver of a medical staff has been described in the study. The results exhibited that variable analysis was able to derive fewer numbers of features from adult, adolescent, and child screening methods yet maintained competitive predictive accuracy, sensitivity, and specificity rates | |||||||||||||||
Risk of Bias |
|
Paper 14
aper Name: Chronic obstructive lung disease "expert system": validation of a predictive tool for assisting diagnosis
Authors or developers | Braido, F. Santus, P. Corsico, A. G. Di Marco, F. Melioli, G. Scichilone, N. Solidoro, P. | |||||||||||||||
---|---|---|---|---|---|---|---|---|---|---|---|---|---|---|---|---|
Year of Publication | 2018 | |||||||||||||||
Full reference of the study | Braido, F., Santus, P., Corsico, A. G., Di Marco, F., Melioli, G., Scichilone, N., & Solidoro, P. (2018). Chronic obstructive lung disease ‘expert system’: validation of a predictive tool for assisting diagnosis. International journal of chronic obstructive pulmonary disease, 13, 1747. | |||||||||||||||
Abstract | Purpose: The purposes of this study were development and validation of an expert system (ES) aimed at supporting the diagnosis of chronic obstructive lung disease (COLD). Methods: A questionnaire and a WebFlex code were developed and validated in silico. An expert panel pilot validation on 60 cases and a clinical validation on 241 cases were performed. Results: The developed questionnaire and code validated in silico resulted in a suitable tool to support the medical diagnosis. The clinical validation of the ES was performed in an academic setting that included six different reference centers for respiratory diseases. The results of the ES expressed as a score associated with the risk of suffering from COLD were matched and compared with the final clinical diagnoses. A set of 60 patients were evaluated by a pilot expert panel validation with the aim of calculating the sample size for the clinical validation study. The concordance analysis between these preliminary ES scores and diagnoses performed by the experts indicated that the accuracy was 94.7% when both experts and the system confirmed the COLD diagnosis and 86.3% when COLD was excluded. Based on these results, the sample size of the validation set was established in 240 patients. The clinical validation, performed on 241 patients, resulted in ES accuracy of 97.5%, with confirmed COLD diagnosis in 53.6% of the cases and excluded COLD diagnosis in 32% of the cases. In 11.2% of cases, a diagnosis of COLD was made by the experts, although the imaging results showed a potential concomitant disorder. Conclusion: The ES presented here (COLDES) is a safe and robust supporting tool for COLD diagnosis in primary care settings. | |||||||||||||||
Country of Research | Italy | |||||||||||||||
Design of Study | Unclear | |||||||||||||||
Duration of Study | Not specified | |||||||||||||||
Name of Condition | Chronic obstructive lung disease | |||||||||||||||
Artificial Intelligence Technique Used | The expert system is based on frame rules (representing the knowledge base) driving the system itself and on forms for input and output. Coded in WebFlex., The expert system was developed on the basis of rules developed by expert pulmonologists by using specific symptomatic pattern, lung function, X-ray. | |||||||||||||||
Providers’ involvement in | Developing : Not specified,Testing : Pulmonologists,Validating : General physicians, pulmonologists | |||||||||||||||
Accuracy of the AI Intervention | Training (true positive , system + expert): 94.7%, True negative()system + expert): 86.3%, Validation: True positive 97.5% expert, True negative: 53.6%), excluded cases of chronic obstructive lung disease: 32% | |||||||||||||||
Patient-related Outcomes Assessed | Not specified | |||||||||||||||
Primary Healthcare Worker Related Outcomes Assessed | Not specified | |||||||||||||||
Healthcare System-related Outcomes Assessed | Not specified | |||||||||||||||
Reached Target Population? | Yes : The expert system identified patients with chronic obstructive lung disease | |||||||||||||||
Adoption | Yes (number of providers i.e. PHC participating) : Not specified | |||||||||||||||
Implementation | The study was applied in a clinical setting and validated by expert pulmonologists | |||||||||||||||
Maintenance | No | |||||||||||||||
Key Conclusions | The expert system is a safe and robust supporting tool for chronic obstructive lung disease diagnosis in primary care settings | |||||||||||||||
Risk of Bias |
|
Paper 15
Paper Name: A machine learning based approach to identify protected health information in Chinese clinical text
Authors or developers | Du, L. Xia, C. Deng, Z. Lu, G. Xia, S. Ma, J. | |||||||||||||||
---|---|---|---|---|---|---|---|---|---|---|---|---|---|---|---|---|
Year of Publication | 2018 | |||||||||||||||
Full reference of the study | Du, L., Xia, C., Deng, Z., Lu, G., Xia, S., & Ma, J. (2018). A machine learning based approach to identify protected health information in Chinese clinical text. International journal of medical informatics, 116, 24-32. | |||||||||||||||
Abstract | BACKGROUND: With the increasing application of electronic health records (EHRs) in the world, protecting private information in clinical text has drawn extensive attention from healthcare providers to researchers. De-identification, the process of identifying and removing protected health information (PHI) from clinical text, has been central to the discourse on medical privacy since 2006. While de-identification is becoming the global norm for handling medical records, there is a paucity of studies on its application on Chinese clinical text. Without efficient and effective privacy protection algorithms in place, the use of indispensable clinical information would be confined. OBJECTIVES: We aimed to (i) describe the current process for PHI in China, (ii) propose a machine learning based approach to identify PHI in Chinese clinical text, and (iii) validate the effectiveness of the machine learning algorithm for de-identification in Chinese clinical text. METHODS: Based on 14,719 discharge summaries from regional health centers in Ya’an City, Sichuan province, China, we built a conditional random fields (CRF) model to identify PHI in clinical text, and then used the regular expressions to optimize the recognition results of the PHI categories with fewer samples. RESULTS: We constructed a Chinese clinical text corpus with PHI tags through substantial manual annotation, wherein the descriptive statistics of PHI manifested its wide range and diverse categories. The evaluation showed with a high F-measure of 0.9878 that our CRF-based model had a good performance for identifying PHI in Chinese clinical text. CONCLUSION: The rapid adoption of EHR in the health sector has created an urgent need for tools that can parse patient specific information from Chinese clinical text. Our application of CRF algorithms for de-identification has shown the potential to meet this need by offering a highly accurate and flexible solution to analyzing Chinese clinical text. | |||||||||||||||
Country of Research | China | |||||||||||||||
Design of Study | Unclear : Not described but a cohort study in reviewer’s opinion | |||||||||||||||
Duration of Study | Not specified | |||||||||||||||
Name of Condition | Not applicable | |||||||||||||||
Artificial Intelligence Technique Used | Conditional random fields, Chinese clinical text corpus with protected health information (PHI) tags via substantial manual annotation and also ran the descriptive statistics of protected health information in corpus from level-specific (Tertiary, Secondary, and Primary) medical institutions Jieba tool: a tool that splits Chinese text into a sequence of words according to the predefined word segmentation rules Medical aDictionaries used: ICD-10 (the International Classification of Disease, 10th Revision) and surgery operation concepts in ICD-9-CM-3 (the International Classification of Diseases, 9th Revision, Clinical Modification, 3th Revision) Lexical features: Lexical features contain the current token and its part of speech (POS) tag, four previous and four next tokens and their corresponding POS tags, which are always a helpful indicator for identifying the boundaries of PHI. For example, prepositions such as “at” and “to” often appear in front of LOCATION and INSTITUTION entities. Dictionary features: We extracted the PHI entities of PROVINCE, CITY, COUNTY, and INSTITUTION in the training data and affixed them with webpages of province, city, county, and medical institution to generate the dictionaries, wherein all of the elements, rather than the phrases, are taken as tokens. The full names and abbreviations of 34 provinces in China, 21 cities, 181 counties in Sichuan and 212 medical institutions in Sichuan ya’an were incorporated in the PROVINCE, CITY, COUNTY and INSTITUTION dictionaries, respectively, to facilitate further classification of PHI entities. | |||||||||||||||
Providers’ involvement in | Developing : Not specified,Testing : Not specified,Validating : Not specified | |||||||||||||||
Accuracy of the AI Intervention | Precesion [Conditional random field + Lexicon: 98.5%, Conditional random field + Lexicon + dictionary: 99.27%, Conditional random field + rules: 99.27%], Recall [Conditional random field + Lexicon: 96.96%, Conditional random field + Lexicon + dictionary: 98.19%, Conditional random field + rules: 98.29%], F-measure [Conditional random field + Lexicon: 97.73%, Conditional random field + Lexicon + dictionary: 98.73%, Conditional random field + rules: 98.78%] | |||||||||||||||
Patient-related Outcomes Assessed | Not specified | |||||||||||||||
Primary Healthcare Worker Related Outcomes Assessed | Not specified | |||||||||||||||
Healthcare System-related Outcomes Assessed | Not specified | |||||||||||||||
Reached Target Population? | Yes | |||||||||||||||
Adoption | Yes (number of providers i.e. PHC participating) : 180 primary care institutions participated in Ya’an city | |||||||||||||||
Implementation | The data of 14719 discharfe summaries from regional health information platform (180: primary care institutions, 30: Secondary care institutions, 2: tertiary care institutions.) in Ya’an City, Sichuan | |||||||||||||||
Maintenance | Not specified | |||||||||||||||
Key Conclusions | The rapid adoption of electronic health record in the health sector has created an urgent need for tools that can parse patient specific information from Chinese clinical text. Our application of conditional random fields algorithms for de-identification has shown the potential to meet this need by offering a highly accurate and flexible solution to analyzing Chinese clinical text. | |||||||||||||||
Risk of Bias |
|
Paper 16
Paper Name: Automatic address validation and health record review to identify homeless Social Security disability applicants
Authors or developers | Erickson, J. Abbott, K. Susienka, L. | |||||||||||||||
---|---|---|---|---|---|---|---|---|---|---|---|---|---|---|---|---|
Year of Publication | 2018 | |||||||||||||||
Full reference of the study | Jennifer Erickson, Kenneth Abbott, Lucinda Susienka, Automatic address validation and health record review to identify homeless Social Security disability applicants, Journal of Biomedical Informatics, Volume 82, 2018, Pages 41-46, ISSN 1532-0464, https://doi.org/10.1016/j.jbi.2018.04.012. | |||||||||||||||
Abstract | OBJECTIVE: Homeless patients face a variety of obstacles in pursuit of basic social services. Acknowledging this, the Social Security Administration directs employees to prioritize homeless patients and handle their disability claims with special care. However, under existing manual processes for identification of homelessness, many homeless patients never receive the special service to which they are entitled. In this paper, we explore address validation and automatic annotation of electronic health records to improve identification of homeless patients. MATERIALS AND METHODS: We developed a sample of claims containing medical records at the moment of arrival in a single office. Using address validation software, we reconciled patient addresses with public directories of homeless shelters, veterans’ hospitals and clinics, and correctional facilities. Other tools annotated electronic health records. We trained random forests to identify homeless patients and validated each model with 10-fold cross validation. RESULTS: For our finished model, the area under the receiver operating characteristic curve was 0.942. The random forest improved sensitivity from 0.067 to 0.879 but decreased positive predictive value to 0.382. DISCUSSION: Presumed false positive classifications bore many characteristics of homelessness. Organizations could use these methods to prompt early collection of information necessary to avoid labor-intensive attempts to reestablish contact with homeless individuals. Annually, such methods could benefit tens of thousands of patients who are homeless, destitute, and in urgent need of assistance. CONCLUSION: We were able to identify many more homeless patients through a combination of automatic address validation and natural language processing of unstructured electronic health records. | |||||||||||||||
Country of Research | USA | |||||||||||||||
Design of Study | Qualitative study | |||||||||||||||
Duration of Study | 1 year | |||||||||||||||
Name of Condition | Homeless Social Security disability | |||||||||||||||
Artificial Intelligence Technique Used | Natural language processing | |||||||||||||||
Providers’ involvement in | Developing : na,Testing : na,Validating : na | |||||||||||||||
Accuracy of the AI Intervention | 0.942, Random forest improved sensitivity: 0.067 to 0.879, Positive predictive value: 0.382 | |||||||||||||||
Patient-related Outcomes Assessed | A combination of automatic address validation and health record review can help identify homeless disability applicants | |||||||||||||||
Primary Healthcare Worker Related Outcomes Assessed | na | |||||||||||||||
Healthcare System-related Outcomes Assessed | na | |||||||||||||||
Reached Target Population? | Yes : Social service agencies may benefit from implementation of similar methods. | |||||||||||||||
Adoption | Yes (number of providers i.e. PHC participating) : Minnesota Disability Determination Services | |||||||||||||||
Implementation | not specified | |||||||||||||||
Maintenance | Not specified | |||||||||||||||
Key Conclusions | Authors were able to identify homeless patients other than reported through a combination of automatic address validation and natural language processing of unstructured electronic health records. | |||||||||||||||
Risk of Bias |
|
Paper 17
Paper Name: Quantifying the incidence and burden of herpes zoster in New Zealand general practice: a retrospective cohort study using a natural language processing software inference algorithm
Authors or developers | Turner, N. M. MacRae, J. Nowlan, M. L. McBain, L. Stubbe, M. H. Dowell, A. | |||||||||||||||
---|---|---|---|---|---|---|---|---|---|---|---|---|---|---|---|---|
Year of Publication | 2018 | |||||||||||||||
Full reference of the study | Turner NM, MacRae J, Nowlan ML, et al. Quantifying the incidence and burden of herpes zoster in New Zealand general practice: a retrospective cohort study using a natural language processing software inference algorithm. BMJ Open 2018;8:e021241. doi:10.1136/ bmjopen-2017-021241 | |||||||||||||||
Abstract | OBJECTIVE: To investigate the incidence of primary care presentations for herpes zoster (zoster) in a representative New Zealand population and to evaluate the utilisation of primary healthcare services following zoster diagnosis. DESIGN: A cross-sectional retrospective cohort study used a natural language processing software inference algorithm to identify general practice consultations for zoster by interrogating 22million electronic medical record (EMR) transactions routinely recorded from January 2005 to December 2015. Data linking enabled analysis of the demographics of each case. The frequency of doctor visits was assessed prior to and after the first consultation diagnosing zoster to determine health service utilisation. SETTING: General practice, using EMRs from two primary health organisations located in the lower North Island, New Zealand. PARTICIPANTS: Thirty-nine general practices consented interrogation of their EMRs to access deidentified records for all enrolled patients. Out-of-hours and practice nurse consultations were excluded. MAIN OUTCOME MEASURES: The incidence of first and repeated zoster-related visits to the doctor across all age groups and associated patient demographics. To determine whether zoster affects workload in general practice. RESULTS: Overall, for 6 189 019 doctor consultations, the incidence of zoster was 48.6 per 10000 patient-years (95%CI 47.6 to 49.6). Incidence increased from the age of 50 years to a peak rate of 128 per 10000 in the age group of 80-90 years and was significantly higher in females than males (p<0.001). Over this 11-year period, incidence increased gradually, notably in those aged 80-85 years. Only 19% of patients had one or more follow-up zoster consultations within 12 months of a zoster index consultation. The frequency of consultations, for any reason, did not change between periods before and after the diagnosis. CONCLUSIONS: Zoster consultations in general practice are rare, and the burden of these cases on overall general practice caseload is low. | |||||||||||||||
Country of Research | New Zealand | |||||||||||||||
Design of Study | Cohort study,Unclear (Cross-sectional) | |||||||||||||||
Duration of Study | 10 years,(January 2005 to December 2015) | |||||||||||||||
Name of Condition | Herpes zoster | |||||||||||||||
Artificial Intelligence Technique Used | Natural language processing software inference algorithm to identify herpes zoster (zoster) presentation rates and service utilisation using primary care electronic medical records over an 11-year period | |||||||||||||||
Provider’s involvement in | Developing : Not specified,Testing : Not specified,Validating : Not specified | |||||||||||||||
Accuracy of the AI Intervention | The natural language algorithm had a positive predictive value of 0.82 (95% CI 0.72 to 0.92), specificity of 0.9998 (95% CI 0.9997 to 0.9999) and sensitivity of 0.84 (95% CI 0.74 to 0.92). This was more accurate than using keywords only (positive predictive value:0.66, specificity 0.9994 and sensitivity 1.0) or using a single clinical expert (positive predictive value:0.53, specificity 0.9991 and sensitivity 0.93). | |||||||||||||||
Patient-related Outcomes Assessed | Despite a low frequency of zoster cases, the large data set enabled analysis of rates of zoster incidence by age bands and different demographics across the whole time period. The algorithm was designed in study to maximise specificity and accuracy, thereby generating a conservative estimate of the burden of zoster presentations in primary care by keeping false positives to a minimum. | |||||||||||||||
Primary Healthcare Worker Related Outcomes Assessed | The overall age-adjusted apparent rate of zoster index consultations was 42.7 per 10000 person-years observed (95%CI 41.9 to 43.5), with an estimated true rate of 48.6 (95% CI 47.6 to 49.6). There were 10316 index consultations for zoster and 3060 zoster-related follow-up consultations. The apparent rate for zoster index consultations was 16.7 per 10000 doctor consultations (95%CI 16.3 to 17.0) with an estimated true rate of 17.5 (95% CI 17.1 to 17.9). This was the equivalent to one in 571 doctor consultations. The rate of consultations were much higher in older age groups, as shown in, with the highest rate in the age group of 80�90years at 128 consultations per 10000 person-years. | |||||||||||||||
Healthcare System-related Outcomes Assessed | Not specified | |||||||||||||||
Reached Target Population? | Yes | |||||||||||||||
Adoption | Yes (number of providers i.e. PHC participating) : 39 general practices,Not specified | |||||||||||||||
Implementation | Not specified | |||||||||||||||
Maintenance | No | |||||||||||||||
Key Conclusions | The study concludes that the natural language processing algorithm had higher accuracy than using keyword or a single clinical expert. Moreover, the study reveals that Zoster consultations in general practice are rare, and the burden of these cases on overall general practice caseload is low. | |||||||||||||||
Risk of Bias |
|
Paper 18
Paper Name: Methods for estimating kidney disease stage transition probabilities using electronic medical records
Authors or developers | Luo, L. Small, D. Stewart, W. F. Roy, J. A. | |||||||||||||||
---|---|---|---|---|---|---|---|---|---|---|---|---|---|---|---|---|
Year of Publication | 2013 | |||||||||||||||
Full reference of the study | Luo, Lola, et al. “Methods for estimating kidney disease stage transition probabilities using electronic medical records.” eGEMs 1.3 (2013). | |||||||||||||||
Abstract | Chronic diseases are often described by stages of severity. Clinical decisions about what to do are influenced by the stage, whether a patient is progressing, and the rate of progression. For chronic kidney disease (CKD), relatively little is known about the transition rates between stages. To address this, we used electronic health records (EHR) data on a large primary care population, which should have the advantage of having both sufficient follow-up time and sample size to reliably estimate transition rates for CKD. However, EHR data have some features that threaten the validity of any analysis. In particular, the timing and frequency of laboratory values and clinical measurements are not determined a priori by research investigators, but rather, depend on many factors, including the current health of the patient. We developed an approach for estimating CKD stage transition rates using hidden Markov models (HMMs), when the level of information and observation time vary among individuals. To estimate the HMMs in a computationally manageable way, we used a “discretization” method to transform daily data into intervals of 30 days, 90 days, or 180 days. We assessed the accuracy and computation time of this method via simulation studies. We also used simulations to study the effect of informative observation times on the estimated transition rates. Our simulation results showed good performance of the method, even when missing data are non-ignorable. We applied the methods to EHR data from over 60,000 primary care patients who have chronic kidney disease (stage 2 and above). We estimated transition rates between six underlying disease states. The results were similar for men and women. | |||||||||||||||
Country of Research | USA | |||||||||||||||
Design of Study | Unclear | |||||||||||||||
Duration of Study | 6 years, 6 months (July 30th, 2003 to Dec. 31st, 2009) | |||||||||||||||
Name of Condition | Kidney Disease Stage Transition | |||||||||||||||
Artificial Intelligence Technique Used | Hidden Markov models, Missing data mechanism (Missing at random) generated as an indicator for variable for missing data. | |||||||||||||||
Provider’s involvement in | Developing : Not specified,Testing : Not specified,Validating : Not specified | |||||||||||||||
Accuracy of the AI Intervention | Not specified, For the missing data mechanism the absolute bias is 0.007 in the 30-day interval, 0.013 in the 90-day interval, and 0.023 in the 180-day interval. | |||||||||||||||
Patient-related Outcomes Assessed | Over 60,000 primary care patients who have chronic kidney disease (stage 2 and above) data was analysed. The estimated transition rates between six underlying disease states were computed. The results were similar for men and women. | |||||||||||||||
Primary Healthcare Worker Related Outcomes Assessed | Not specified | |||||||||||||||
Healthcare System-related Outcomes Assessed | Not specified | |||||||||||||||
Reached Target Population? | Yes | |||||||||||||||
Adoption | Not specified | |||||||||||||||
Implementation | Not specified | |||||||||||||||
Maintenance | Not specified (Unclear) | |||||||||||||||
Key Conclusions | Over 60,000 primary care patients who have chronic kidney disease (stage 2 and above) data was analysed. The estimated transition rates between six underlying disease states were computed. The results were similar for men and women. | |||||||||||||||
Risk of Bias |
|
Paper 19
Paper Name: Enabling Stroke Rehabilitation in Home and Community Settings: A Wearable Sensor-Based Approach for Upper-Limb Motor Training
Authors or developers | Lee, S. I. Adans-Dester, C. P. Grimaldi, M. Dowling, A. V. Horak, P. C. Black-Schaffer, R. M. Bonato, P. Gwin, J. T. | |||||||||||||||
---|---|---|---|---|---|---|---|---|---|---|---|---|---|---|---|---|
Year of Publication | 2018 | |||||||||||||||
Full reference of the study | Enabling Stroke Rehabilitation in Home and Community Settings: A Wearable Sensor-Based Approach for Upper-Limb Motor Training | |||||||||||||||
Abstract | High-dosage motor practice can significantly contribute to achieving functional recovery after a stroke. Performing rehabilitation exercises at home and using, or attempting to use, the stroke-affected upper limb during Activities of Daily Living (ADL) are effective ways to achieve high-dosage motor practice in stroke survivors. This paper presents a novel technological approach that enables 1) detecting goal-directed upper limb movements during the performance of ADL, so that timely feedback can be provided to encourage the use of the affected limb, and 2) assessing the quality of motor performance during in-home rehabilitation exercises so that appropriate feedback can be generated to promote high-quality exercise. The results herein presented show that it is possible to detect 1) goal-directed movements during the performance of ADL with a [Formula: see text]-statistic of 87.0% and 2) poorly performed movements in selected rehabilitation exercises with an [Formula: see text]-score of 84.3%, thus enabling the generation of appropriate feedback. In a survey to gather preliminary data concerning the clinical adequacy of the proposed approach, 91.7% of occupational therapists demonstrated willingness to use it in their practice, and 88.2% of stroke survivors indicated that they would use it if recommended by their therapist. | |||||||||||||||
Country of Research | USA | |||||||||||||||
Design of Study | Unclear | |||||||||||||||
Duration of Study | Not specified | |||||||||||||||
Name of Condition | Stroke | |||||||||||||||
Artificial Intelligence Technique Used | Logistic regression classification model | |||||||||||||||
Providers’ involvement in | Developing : Not specified,Testing : Occupational therapist,Validating : Occupational therapist | |||||||||||||||
Accuracy of the AI Intervention | 87% AUC, True positive rate: 79%, True negative rate: 78%. | |||||||||||||||
Patient-related Outcomes Assessed | 88.2% of stroke survivors indicated that they would use it | |||||||||||||||
Primary Healthcare Worker Related Outcomes Assessed | 91.7% of occupational therapists demonstrated willingness to use it | |||||||||||||||
Healthcare System-related Outcomes Assessed | Not specified | |||||||||||||||
Reached Target Population? | Yes | |||||||||||||||
Adoption | Yes (number of providers i.e. PHC participating) : Occupational therapists | |||||||||||||||
Implementation | Not specified | |||||||||||||||
Maintenance | Not specified | |||||||||||||||
Key Conclusions | The authors presented a novel technological approach that utilizes two wearable sensors for detecting goal directed movements during activities of daily living and for determining appropriate feedback during in-home rehabilitation exercise | |||||||||||||||
Risk of Bias |
|
Paper 20
Paper Name: External validation of ADO, DOSE, COTE and CODEX at predicting death in primary care patients with COPD using standard and machine learning approaches
Authors or developers | Morales, D. R. Flynn, R. Zhang, J. Trucco, E. Quint, J. K. Zutis, K. | |||||||||||||||
---|---|---|---|---|---|---|---|---|---|---|---|---|---|---|---|---|
Year of Publication | 2018 | |||||||||||||||
Full reference of the study | Morales, D., Flynn, R., Zhang, J., Trucco, E., & Quint, J. K. (2018). External validation of ADO, DOSE, COTE and CODEX at predicting death in primary care patients with COPD using standard and machine learning approaches. Respiratory Medicine, 138, 150-155. https://doi.org/10.1016/j.rmed.2018.04.003 | |||||||||||||||
Abstract | BACKGROUND: Several models for predicting the risk of death in people with chronic obstructive pulmonary disease (COPD) exist but have not undergone large scale validation in primary care. The objective of this study was to externally validate these models using statistical and machine learning approaches. METHODS: We used a primary care COPD cohort identified using data from the UK Clinical Practice Research Datalink. Age-standardised mortality rates were calculated for the population by gender and discrimination of ADO (age, dyspnoea, airflow obstruction), COTE (COPD-specific comorbidity test), DOSE (dyspnoea, airflow obstruction, smoking, exacerbations) and CODEX (comorbidity, dyspnoea, airflow obstruction, exacerbations) at predicting death over 1-3 years measured using logistic regression and a support vector machine learning (SVM) method of analysis. RESULTS: The age-standardised mortality rate was 32.8 (95%CI 32.5-33.1) and 25.2 (95%CI 25.4-25.7) per 1000 person years for men and women respectively. Complete data were available for 54879 patients to predict 1-year mortality. ADO performed the best (c-statistic of 0.730) compared with DOSE (c-statistic 0.645), COTE (c-statistic 0.655) and CODEX (c-statistic 0.649) at predicting 1-year mortality. Discrimination of ADO and DOSE improved at predicting 1-year mortality when combined with COTE comorbidities (c-statistic 0.780 ADO + COTE; c-statistic 0.727 DOSE + COTE). Discrimination did not change significantly over 1-3 years. Comparable results were observed using SVM. CONCLUSION: In primary care, ADO appears superior at predicting death in COPD. Performance of ADO and DOSE improved when combined with COTE comorbidities suggesting better models may be generated with additional data facilitated using novel approaches. | |||||||||||||||
Country of Research | UK | |||||||||||||||
Design of Study | Cohort study | |||||||||||||||
Duration of Study | 14 years, 01.01.2000 to 01.04.2014 | |||||||||||||||
Name of Condition | Chronic obstructive pulmonary disease | |||||||||||||||
Artificial Intelligence Technique Used | Logistic regression and a support vector 12 machine learning (SVM) | |||||||||||||||
Providers’ involvement in | Developing : Not specified,Testing : Not specified,Validating : Not specified | |||||||||||||||
Accuracy of the AI Intervention | ADO index score 27 performed the best with a 1 year c-statistic of 0.723 1 year DOSE index score 8 1 c-statistic of 0.654 COTE index score of 0.650 CODEX index score of 0.651, Class weighting was used to improve prediction accuracy and 95% confidence intervals were 14 generated through bootstrapping | |||||||||||||||
Patient-related Outcomes Assessed | The age-standardised mortality rate was 32.8 (95%CI 32.5-33.1) and 25.2 (95%CI 14 25.4-25.7) per 1000 person years for men and women respectively. Complete data were 15 available for 54879 patients to predict 1-year mortality | |||||||||||||||
Primary Healthcare Worker Related Outcomes Assessed | Not specified | |||||||||||||||
Healthcare System-related Outcomes Assessed | Not specified | |||||||||||||||
Reached Target Population? | Yes | |||||||||||||||
Adoption | Not specified | |||||||||||||||
Implementation | Our study has shown that predictive 21 performance can be improved by incorporating more clinical information, in this example 22 using COTE comorbidities. However, incorporating larger amounts of data may be infeasible 23 to interpret through human factors alone. Using a limited feature set, SVM performs as well 24 as standard logistic regression helping to validate this approach which could now be applied 25 to a large data set that includes far more clinical data (such as blood results, prescriptions, 26 pattern of health care access and social care data). | |||||||||||||||
Maintenance | No | |||||||||||||||
Key Conclusions | Complete data were 15 available for 54879 patients to predict 1-year mortality. ADO performed the best (c-statistic 16 of 0.730) compared with DOSE (c-statistic 0.645), COTE (c-statistic 0.655) and CODEX (c17 statistic 0.649) at predicting 1-year mortality. Discrimination of ADO and DOSE improved 18 discrimination at predicting 1-year mortality when combined with COTE comorbidities (c19 statistic 0.780 ADO+COTE; c-statistic 0.727 DOSE+COTE). Discrimination did not change 20 significantly over 1-3 years. Comparable results were observed using support vector 12 machine learning (SVM) method of analysis. In primary care, ADO appears superior at predicting death in COPD. Performance of ADO and DOSE improved when combined with COTE comorbidities suggesting better models may be generated with additional data facilitated using novel approaches. | |||||||||||||||
Risk of Bias |
|
Paper 21
Paper Name: Automatic infection detection based on electronic medical records
Authors or developers | Tou, H. Yao, L. Wei, Z. Zhuang, X. Zhang, B. | |||||||||||||||
---|---|---|---|---|---|---|---|---|---|---|---|---|---|---|---|---|
Year of Publication | 2018 | |||||||||||||||
Full reference of the study | Tou, Huaixiao, et al. “Automatic infection detection based on electronic medical records.” BMC bioinformatics 19.5 (2018): 117. | |||||||||||||||
Abstract | BACKGROUND: Making accurate patient care decision, as early as possible, is a constant challenge, especially for physicians in the emergency department. The increasing volumes of electronic medical records (EMRs) open new horizons for automatic diagnosis. In this paper, we propose to use machine learning approaches for automatic infection detection based on EMRs. Five categories of information are utilized for prediction, including personal information, admission note, vital signs, diagnose test results and medical image diagnose. RESULTS: Experimental results on a newly constructed EMRs dataset from emergency department show that machine learning models can achieve a decent performance for infection detection with area under the receiver operator characteristic curve (AUC) of 0.88. Out of all the five types of information, admission note in text form makes the most contribution with the AUC of 0.87. CONCLUSIONS: This study provides a state-of-the-art EMRs processing system to automatically make medical decisions. It extracts five types of features associated with infection and achieves a decent performance on automatic infection detection based on machine learning models. | |||||||||||||||
Country of Research | China | |||||||||||||||
Design of Study | Cohort study | |||||||||||||||
Duration of Study | 4 year, 2012-2016 | |||||||||||||||
Name of Condition | Infection, cess, Necrosis, Gangrene, Pyogenic, Sepsis, Erysipelatous, Pneumonia, Pyothorax, Mastitis, Perforation, Peritonitis, Acute cholecystitis, Gangrenous cholecystitis, Acute attacking of chronic cholecystitis, Acute cholangitis, Acute suppurative cholangitis, Acute gangrenous cholangitis, Biliary pancreatitis, Acute appendicitis, Acute suppurated appendicitis, Acute gangrened appendicitis, Acute purulent gangrenous appendicitis, Acute phlegmonous appendicitis, Systemic inflammatory response syndrome, Sepsis, Septic shock, Acute attacking of chronic appendicitis | |||||||||||||||
Artificial Intelligence Technique Used | Machine learning: Random forest, logistic regression CV, Bernoulli NB, Gradient boosting classifier | |||||||||||||||
Providers’ involvement in | Developing : Not specified,Testing : Not specified,Validating : Not specified | |||||||||||||||
Accuracy of the AI Intervention | Random forest: 0.84, logistic regression CV: 0.87, Bernoulli NB: 0.68, Gradient boosting classifier: 0.88 | |||||||||||||||
Patient-related Outcomes Assessed | Not specified | |||||||||||||||
Primary Healthcare Worker Related Outcomes Assessed | Not specified | |||||||||||||||
Healthcare System-related Outcomes Assessed | Not specified | |||||||||||||||
Reached Target Population? | Yes | |||||||||||||||
Adoption | Not specified | |||||||||||||||
Implementation | Not implemented studies on a cohort of electronic medical health record. | |||||||||||||||
Maintenance | No | |||||||||||||||
Key Conclusions | The study demonstrates a state-of-the-art electronic medical records processing system to automatically make medical decision. The single factor correlation analysis shows the processing system is able to identify indicative factors for the detection of infection. Research also analyze the effectiveness of different types of features for infection detection and reveal the effectiveness of text-based features. The system, using all features achieves the best performance with AUC over 88%. | |||||||||||||||
Risk of Bias |
|
Paper 22
Paper Name: Detecting Motor Impairment in Early Parkinson's Disease via Natural Typing Interaction With Keyboards: Validation of the neuroQWERTY Approach in an Uncontrolled At-Home Setting
Authors or developers | Arroyo-Gallego, T. Ledesma-Carbayo, M. J. Butterworth, I. Matarazzo, M. Montero-Escribano, P. Puertas-Martin, V. Gray, M. L. Giancardo, L. Sanchez-Ferro, A. | |||||||||||||||
---|---|---|---|---|---|---|---|---|---|---|---|---|---|---|---|---|
Year of Publication | 2018 | |||||||||||||||
Full reference of the study | Arroyo-Gallego, Teresa, et al. “Detecting motor impairment in early Parkinson’s disease via natural typing interaction with keyboards: validation of the neuroQWERTY approach in an uncontrolled at-home setting.” Journal of medical Internet research 20.3 (2018): e89. | |||||||||||||||
Abstract | BACKGROUND: Parkinson’s disease (PD) is the second most prevalent neurodegenerative disease and one of the most common forms of movement disorder. Although there is no known cure for PD, existing therapies can provide effective symptomatic relief. However, optimal titration is crucial to avoid adverse effects. Today, decision making for PD management is challenging because it relies on subjective clinical evaluations that require a visit to the clinic. This challenge has motivated recent research initiatives to develop tools that can be used by nonspecialists to assess psychomotor impairment. Among these emerging solutions, we recently reported the neuroQWERTY index, a new digital marker able to detect motor impairment in an early PD cohort through the analysis of the key press and release timing data collected during a controlled in-clinic typing task. OBJECTIVE: The aim of this study was to extend the in-clinic implementation to an at-home implementation by validating the applicability of the neuroQWERTY approach in an uncontrolled at-home setting, using the typing data from subjects’ natural interaction with their laptop to enable remote and unobtrusive assessment of PD signs. METHODS: We implemented the data-collection platform and software to enable access and storage of the typing data generated by users while using their computer at home. We recruited a total of 60 participants; of these participants 52 (25 people with Parkinson’s and 27 healthy controls) provided enough data to complete the analysis. Finally, to evaluate whether our in-clinic-built algorithm could be used in an uncontrolled at-home setting, we compared its performance on the data collected during the controlled typing task in the clinic and the results of our method using the data passively collected at home. RESULTS: Despite the randomness and sparsity introduced by the uncontrolled setting, our algorithm performed nearly as well in the at-home data (area under the receiver operating characteristic curve [AUC] of 0.76 and sensitivity/specificity of 0.73/0.69) as it did when used to evaluate the in-clinic data (AUC 0.83 and sensitivity/specificity of 0.77/0.72). Moreover, the keystroke metrics presented a strong correlation between the 2 typing settings, which suggests a minimal influence of the in-clinic typing task in users’ normal typing. CONCLUSIONS: The finding that an algorithm trained on data from an in-clinic setting has comparable performance with that tested on data collected through naturalistic at-home computer use reinforces the hypothesis that subtle differences in motor function can be detected from typing behavior. This work represents another step toward an objective, user-convenient, and quasi-continuous monitoring tool for PD. | |||||||||||||||
Country of Research | Spain | |||||||||||||||
Design of Study | Cohort study,Unclear : Longitudnal study | |||||||||||||||
Duration of Study | 6 months | |||||||||||||||
Name of Condition | Parkinson Disease | |||||||||||||||
Artificial Intelligence Technique Used | neuroQWERTY, nQi is the output of a computational algorithm that uses the information contained in the sequences of hold times | |||||||||||||||
Providers’ involvement in | Developing : Not specified,Testing : Not specified,Validating : Not specified | |||||||||||||||
Accuracy of the AI Intervention | At home (0.76 [0.66-0.88]), In clinic (0.83 [0.74-0.92]) | |||||||||||||||
Patient-related Outcomes Assessed | neuroQWERTY index (nQi) performance comparison Parkinson: Mean (S.D): Clinic: 0.092(0.058), Home: 0.09(0.048) Healthy: Mean (S.D): Clinic: 0.092(0.058), Home: 0.09(0.048) | |||||||||||||||
Primary Healthcare Worker Related Outcomes Assessed | Not specified | |||||||||||||||
Healthcare System-related Outcomes Assessed | Not specified | |||||||||||||||
Reached Target Population? | Yes | |||||||||||||||
Adoption | Not specified | |||||||||||||||
Implementation | Not specified | |||||||||||||||
Maintenance | No | |||||||||||||||
Key Conclusions | This study validated the findings of neuroQWERTY algorithm in a home based setting and validated its findings to be at a comparable performance with the in clinic data. | |||||||||||||||
Risk of Bias |
|
Paper 23
Paper Name: Home Health Care: Nurse-Physician Communication, Patient Severity, and Hospital Readmission
Authors or developers | Pesko, M. F. Gerber, L. M. Peng, T. R. Press, M. J. | |||||||||||||||
---|---|---|---|---|---|---|---|---|---|---|---|---|---|---|---|---|
Year of Publication | 2018 | |||||||||||||||
Full reference of the study | Pesko, Michael F., et al. “Home health care: nurse’physician communication, patient severity, and hospital readmission.” Health services research 53.2 (2018): 1008-1024. | |||||||||||||||
Abstract | OBJECTIVE: To evaluate whether communication failures between home health care nurses and physicians during an episode of home care after hospital discharge are associated with hospital readmission, stratified by patients at high and low risk of readmission. DATA SOURCE/STUDY SETTING: We linked Visiting Nurse Services of New York electronic medical records for patients with congestive heart failure in 2008 and 2009 to hospitalization claims data for Medicare fee-for-service beneficiaries. STUDY DESIGN: Linear regression models and a propensity score matching approach were used to assess the relationship between communication failure and 30-day readmission, separately for patients with high-risk and low-risk readmission probabilities. DATA COLLECTION/EXTRACTION METHODS: Natural language processing was applied to free-text data in electronic medical records to identify failures in communication between home health nurses and physicians. PRINCIPAL FINDINGS: Communication failure was associated with a statistically significant 9.7 percentage point increase in the probability of a patient readmission (32.6 percent of the mean) among high-risk patients. CONCLUSIONS: Poor communication between home health nurses and physicians is associated with an increased risk of hospital readmission among high-risk patients. Efforts to reduce readmissions among this population should consider focusing attention on this factor. | |||||||||||||||
Country of Research | USA | |||||||||||||||
Design of Study | Unclear | |||||||||||||||
Duration of Study | 1 year, 2008-2009 | |||||||||||||||
Name of Condition | Congestive heart faliure | |||||||||||||||
Artificial Intelligence Technique Used | Natural language processing, Linear regression models and a propensity score matching approach | |||||||||||||||
Providers’ involvement in | Developing : Not specified,Testing : Not specified,Validating : Not specified | |||||||||||||||
Accuracy of the AI Intervention | Sensitivity analysis: Analysis 1: 7.9% increased likelihood of readmission due to communication faliure, Analysis 2: 6% increased likelihood of readmission due to communication failure | |||||||||||||||
Patient-related Outcomes Assessed | Patient readmission rate | |||||||||||||||
Primary Healthcare Worker Related Outcomes Assessed | Physician-nurse communication | |||||||||||||||
Healthcare System-related Outcomes Assessed | Not specified | |||||||||||||||
Reached Target Population? | Yes | |||||||||||||||
Adoption | Yes (number of providers i.e. PHC participating) : 778 nurses,Not specified : Nurse: years of experience: One comunication= 7.11 years, S.D= 6.89, all communication= 7.08, SD= 7.22 One communication(Physician: 0.59, Non-surgeon subspecialist:0.35, Surgeon: 0.06) All communication(Physician: 0.51, Non-surgeon subspecialist: 0.41, Surgeon: 0.07) | |||||||||||||||
Implementation | Not implemented, tested on electronic medical records | |||||||||||||||
Maintenance | Not specified | |||||||||||||||
Key Conclusions | The study reports a natural language processing algorithm that from home health care electronic medical records match with medican data examines how faliure in communication between nurses and physicians during an episode in home health care can influence the patients probability for a 30 day re admission. | |||||||||||||||
Risk of Bias |
|
Paper 24
Paper Name: Examining Healthcare Utilization Patterns of Elderly Middle-Aged Adults in the United States
Authors or developers | Zayas, C. E. He, Z. Yuan, J. Maldonado-Molina, M. Hogan, W. Modave, F. Guo, Y. Bian, J. | |||||||||||||||
---|---|---|---|---|---|---|---|---|---|---|---|---|---|---|---|---|
Year of Publication | 2016 | |||||||||||||||
Full reference of the study | Zayas, Cilia E., et al. “Examining Healthcare Utilization Patterns of Elderly and Middle-Aged Adults in the United States.” The Twenty-Ninth International Flairs Conference. 2016. | |||||||||||||||
Abstract | Elderly patients, aged 65 or older, make up 13.5% of the U.S. population, but represent 45.2% of the top 10% of healthcare utilizers, in terms of expenditures. Middle-aged Americans, aged 45 to 64 make up another 37.0% of that category. Given the high demand for healthcare services by the aforementioned population, it is important to identify high-cost users of healthcare systems and, more importantly, ineffective utilization patterns to highlight where targeted interventions could be placed to improve care delivery. In this work, we present a novel multi-level framework applying machine learning (ML) methods (i.e., random forest regression and hierarchical clustering) to group patients with similar utilization profiles into clusters. We use a vector space model to characterize a patient’s utilization profile as the number of visits to different care providers and prescribed medications. We applied the proposed methods using the 2013 Medical Expenditures Panel Survey (MEPS) dataset. We identified clusters of healthcare utilization patterns of elderly and middle-aged adults in the United States, and assessed the general and clinical characteristics associated with these utilization patterns. Our results demonstrate the effectiveness of the proposed framework to model healthcare utilization patterns. Understanding of these patterns can be used to guide healthcare policy-making and practice. | |||||||||||||||
Country of Research | USA | |||||||||||||||
Design of Study | Cohort study | |||||||||||||||
Duration of Study | 1 year, 2013 | |||||||||||||||
Name of Condition | Diabetes, cancer, coronary heart disease, angina, heart attack, other heart disease, stroke | |||||||||||||||
Artificial Intelligence Technique Used | Random forest regression, hierarchial clustering | |||||||||||||||
Providers’ involvement in | Developing : presented a novel multi-level framework applying machine learning methods (i.e., random forest regression and hierarchical clustering) to group patients with similar utilization profiles into clusters | |||||||||||||||
Accuracy of the AI Intervention | Prediction performance random forest regression model: r squared: 0.46, NRMSE: 1.68, The Silhouette scores for k=20, 100, and 150 are -0.592, 0.007, and 0.065, respectively | |||||||||||||||
Patient-related Outcomes Assessed | Healthcare Utilization Patterns of Elderly Middle-Aged Adults in the United States | |||||||||||||||
Primary Healthcare Worker Related Outcomes Assessed | not specified | |||||||||||||||
Healthcare System-related Outcomes Assessed | Utilization Patterns of patients with similar utilization profiles into clusters thus helping in better optimized health care system | |||||||||||||||
Reached Target Population? | Yes | |||||||||||||||
Adoption | Not specified | |||||||||||||||
Implementation | Not specified | |||||||||||||||
Maintenance | No | |||||||||||||||
Key Conclusions | This study presented a simple but novel vector space model of patients’ utilization profiles. the evaluations, using the 2013 MEPS dataset, demonstrate the usefulness of the proposed approaches in identifying meaningful utilization patterns of elderly and middle-aged adults in the United States. | |||||||||||||||
Risk of Bias |
|
Paper 25
Paper Name: Data-based Decision Rules to Personalize Depression Follow-up
Authors or developers | Lin, Y. Huang, S. Simon, G. E. Liu, S. | |||||||||||||||
---|---|---|---|---|---|---|---|---|---|---|---|---|---|---|---|---|
Year of Publication | 2018 | |||||||||||||||
Full reference of the study | Lin, Y., Huang, S., Simon, G.E. et al. Data-based Decision Rules to Personalize Depression Follow-up. Sci Rep 8, 5064 (2018). https://doi.org/10.1038/s41598-018-23326-1 | |||||||||||||||
Abstract | Depression is a common mental illness with complex and heterogeneous progression dynamics. Risk grouping of depression treatment population based on their longitudinal patterns has the potential to enable cost-effective monitoring policy design. This paper establishes a rule-based method to identify a set of risk predictive patterns from person-level longitudinal disease measurements by integrating the data transformation, rule discovery and rule evaluation. We further extend the identified rules to create rule-based monitoring strategies to adaptively monitor individuals with different disease severities. We applied the rule-based method on an electronic health record (EHR) dataset of depression treatment population containing person-level longitudinal Patient Health Questionnaire (PHQ)-9 scores for assessing depression severity. 12 risk predictive rules are identified, and the rule-based prognostic model based on identified rules enables more accurate prediction of disease severity than other prognostic models including RuleFit, logistic regression and Support Vector Machine. Two rule-based monitoring strategies outperform the latest PHQ-9 based monitoring strategy by providing higher sensitivity and specificity. The rule-based method can lead to a better understanding of disease dynamics, achieving more accurate prognostics of disease progressions, personalizing follow-up intervals, and designing cost-effective monitoring of patients in clinical practice. | |||||||||||||||
Country of Research | USA | |||||||||||||||
Design of Study | Unclear | |||||||||||||||
Duration of Study | 5 years, 2007-2012 | |||||||||||||||
Name of Condition | Depression | |||||||||||||||
Artificial Intelligence Technique Used | Rule-based prognostic model RuleFit Logistic regression Support vector machine | |||||||||||||||
Providers’ involvement in | Developing : Not specified,Testing : Not specified,Validating : Not specified | |||||||||||||||
Accuracy of the AI Intervention | Rule-based prognostic model 0.83, RuleFit: 0.81, Logistic regression:0.81, support vector machine 0.81 | |||||||||||||||
Patient-related Outcomes Assessed | The rule-based method can lead to a better understanding of disease dynamics, achieving more accurate prognostics of disease progressions, personalizing follow-up intervals, and designing cost-efective monitoring of patients in clinical practice | |||||||||||||||
Primary Healthcare Worker Related Outcomes Assessed | Not specified | |||||||||||||||
Healthcare System-related Outcomes Assessed | Extended use of Electronic Health Record (EHR) provides an abundance of clinical measurements that may help to predict patients’ disease progressions. Leveraging this rich information can accelerate the transition from one-size-fts-all monitoring guidelines to personalized monitoring strategies | |||||||||||||||
Reached Target Population? | Yes | |||||||||||||||
Adoption | Yes (number of providers i.e. PHC participating),Not specified | |||||||||||||||
Implementation | Implemented rule-based method on an electronic health record (EHR) dataset of depression treatment population containing person-level longitudinal Patient Health Questionnaire (PHQ)-9 scores for assessing depression severity. 12 risk predictive rules are identifed, and the rule-based prognostic model based on identifed rules enables more accurate prediction of disease severity than other prognostic models including RuleFit, logistic regression and Support Vector Machine | |||||||||||||||
Maintenance | Not specified | |||||||||||||||
Key Conclusions | The work showed 12 risk predictive rules from a depression treatment population that can segment individuals into risk subgroups based on their longitudinal patterns. Further the work also developed and evaluated adaptive monitoring strategies based on these identifed rules along with established rule-based analytic framework to automatically leverage the sparse, irregular and time-varying measurements in electronic Health Record data to support the monitoring strategy design by integrating the data transformation, rule discovery and rule evaluation. More generally, the proposed method can lead to a better understanding of disease dynamics, more accurate prognostics of disease progressions, and efcient monitoring of a treatment population in clinical practice. | |||||||||||||||
Risk of Bias |
|
Paper 26
Paper Name: A risk score including body mass index, glycated haemoglobin and triglycerides predicts future glycaemic control in people with type 2 diabetes
Authors or developers | Hertroijs, D. F. L. Elissen, A. M. J. Brouwers, Mcgj Schaper, N. C. Kohler, S. Popa, M. C. Asteriadis, S. Hendriks, S. H. Bilo, H. J. Ruwaard, D. | |||||||||||||||
---|---|---|---|---|---|---|---|---|---|---|---|---|---|---|---|---|
Year of Publication | 2018 | |||||||||||||||
Full reference of the study | Hertroijs DFL, Elissen AMJ, Brouwers Martijn C. G. J., et al. A risk score including body mass index, glycated haemoglobin and triglycerides predicts future glycaemic control in people with type 2 diabetes. Diabetes Obes Metab. 2018;20:681’688. https://doi.org/10.1111/dom.13148 | |||||||||||||||
Abstract | AIM: To identify, predict and validate distinct glycaemic trajectories among patients with newly diagnosed type 2 diabetes treated in primary care, as a first step towards more effective patient-centred care. METHODS: We conducted a retrospective study in two cohorts, using routinely collected individual patient data from primary care practices obtained from two large Dutch diabetes patient registries. Participants included adult patients newly diagnosed with type 2 diabetes between January 2006 and December 2014 (development cohort, n=10528; validation cohort, n=3777). Latent growth mixture modelling identified distinct glycaemic 5-year trajectories. Machine learning models were built to predict the trajectories using easily obtainable patient characteristics in daily clinical practice. RESULTS: Three different glycaemic trajectories were identified: (1) stable, adequate glycaemic control (76.5% of patients); (2) improved glycaemic control (21.3% of patients); and (3) deteriorated glycaemic control (2.2% of patients). Similar trajectories could be discerned in the validation cohort. Body mass index and glycated haemoglobin and triglyceride levels were the most important predictors of trajectory membership. The predictive model, trained on the development cohort, had a receiver-operating characteristic area under the curve of 0.96 in the validation cohort, indicating excellent accuracy. CONCLUSIONS: The developed model can effectively explain heterogeneity in future glycaemic response of patients with type 2 diabetes. It can therefore be used in clinical practice as a quick and easy tool to provide tailored diabetes care. | |||||||||||||||
Country of Research | Netherlands | |||||||||||||||
Design of Study | Cohort study,Unclear : Retrospective | |||||||||||||||
Duration of Study | 5 years, January 1, 2009 and December 31, 2014 | |||||||||||||||
Name of Condition | Type 2 diabetes | |||||||||||||||
Artificial Intelligence Technique Used | Latent growth mixture modelling (LGMM), Akaike Information Criterion, Bayesian Information Criterion and the LoMendel-Rubin-likelihood ratio test. | |||||||||||||||
Providers’ involvement in | Developing : Not specified,Testing : Not specified,Validating : Not specified | |||||||||||||||
Accuracy of the AI Intervention | AUC: 0.96 | |||||||||||||||
Patient-related Outcomes Assessed | Not specified | |||||||||||||||
Primary Healthcare Worker Related Outcomes Assessed | Not specified | |||||||||||||||
Healthcare System-related Outcomes Assessed | The developed model can effectively explain heterogeneity in future glycaemic response of patients with type 2 diabetes. It can therefore be used in clinical practice as a quick and easy tool to provide tailored diabetes care. | |||||||||||||||
Reached Target Population? | Yes | |||||||||||||||
Adoption | Yes (number of providers i.e. PHC participating) : 95 primary care practices in Maastricht | |||||||||||||||
Implementation | Three different glycaemic trajectories were identified: (1) stable, adequate glycaemic control (76.5% of patients); (2) improved glycaemic control (21.3% of patients); and (3) deteriorated glycaemic control (2.2% of patients). Similar trajectories could be discerned in the validation cohort. Body mass index and glycated haemoglobin and triglyceride levels were the most important predictors of trajectory membership. The predictive model, trained on the development cohort, had a receiver-operating characteristic area under the curve of 0.96 in the validation cohort, indicating excellent accuracy | |||||||||||||||
Maintenance | No | |||||||||||||||
Key Conclusions | The developed model can effectively explain heterogeneity in future glycaemic response of patients with type 2 diabetes. It can therefore be used in clinical practice as a quick and easy tool to provide tailored diabetes care. | |||||||||||||||
Risk of Bias |
|
Paper 27
Paper Name: Predictive modeling of colorectal cancer using a dedicated pre-processing pipeline on routine electronic medical records
Authors or developers | Kop, R. Hoogendoorn, M. Teije, A. T. Buchner, F. L. Slottje, P. Moons, L. M. Numans, M. E. | |||||||||||||||
---|---|---|---|---|---|---|---|---|---|---|---|---|---|---|---|---|
Year of Publication | 2016 | |||||||||||||||
Full reference of the study | Kop, Reinier, et al. “Predictive modeling of colorectal cancer using a dedicated pre-processing pipeline on routine electronic medical records.” Computers in biology and medicine 76 (2016): 30-38. | |||||||||||||||
Abstract | Over the past years, research utilizing routine care data extracted from Electronic Medical Records (EMRs) has increased tremendously. Yet there are no straightforward, standardized strategies for pre-processing these data. We propose a dedicated medical pre-processing pipeline aimed at taking on many problems and opportunities contained within EMR data, such as their temporal, inaccurate and incomplete nature. The pipeline is demonstrated on a dataset of routinely recorded data in general practice EMRs of over 260,000 patients, in which the occurrence of colorectal cancer (CRC) is predicted using various machine learning techniques (i.e., CART, LR, RF) and subsets of the data. CRC is a common type of cancer, of which early detection has proven to be important yet challenging. The results are threefold. First, the predictive models generated using our pipeline reconfirmed known predictors and identified new, medically plausible, predictors derived from the cardiovascular and metabolic disease domain, validating the pipeline’s effectiveness. Second, the difference between the best model generated by the data-driven subset (AUC 0.891) and the best model generated by the current state of the art hypothesis-driven subset (AUC 0.864) is statistically significant at the 95% confidence interval level. Third, the pipeline itself is highly generic and independent of the specific disease targeted and the EMR used. In conclusion, the application of established machine learning techniques in combination with the proposed pipeline on EMRs has great potential to enhance disease prediction, and hence early detection and intervention in medical practice. | |||||||||||||||
Country of Research | Netherlands | |||||||||||||||
Design of Study | Unclear | |||||||||||||||
Duration of Study | 4 years,(2007-2011) | |||||||||||||||
Name of Condition | Colorectal cancer | |||||||||||||||
Artificial Intelligence Technique Used | CART, Logistic regression, random forest | |||||||||||||||
Provider’s involvement in | Developing : Not specified,Testing : Not specified,Validating : Not specified | |||||||||||||||
Accuracy of the AI Intervention | CART: Age & gender (AUC: 0.83, 95% CI: 0.81-0.84), Bristol-Birmingham equation (AUC: 0.85, 95%CI: 0.83-0.86) Logistic regression: Age & gender (AUC:0.83 , 95% CI: 0.82-0.85), Bristol-Birmingham equation (AUC: 0.86, 95%CI: 0.85-0.87) Random forest: Age & gender (AUC: 0.83, 95% CI: 0.82-0.84), Bristol-Birmingham equation (AUC: 0.88, 95%CI: 0.87-0.89) | |||||||||||||||
Patient-related Outcomes Assessed | Application of established machine learning techniques in combination with the proposed pipeline on EMRs has great potential to enhance disease prediction, and hence early detection and intervention in medical practice | |||||||||||||||
Primary Healthcare Worker Related Outcomes Assessed | Not specified | |||||||||||||||
Healthcare System-related Outcomes Assessed | Not specified | |||||||||||||||
Reached Target Population? | Yes | |||||||||||||||
Adoption | No | |||||||||||||||
Implementation | Result demonstrated on a dataset of routinely recorded data in general practice EMRs of over 260,000 patients, in which the occurrence of colorectal cancer (CRC) is predicted using various machine learning techniques (i.e., CART, LR, RF) and subsets of the data. CRC is a common type of cancer, of which early detection has proven to be important yet challenging. | |||||||||||||||
Maintenance | No | |||||||||||||||
Key Conclusions | The authors describes pipelines using three machine learning approaches i.e. random forest, CART, logistic regression and reports a great potential for enhacning the prediction of colorectal cancer and its detection., Main results: 1. The predictive models generated using our pipeline reconfirmed known predictors and identified new, medically plausible, predictors derived from the cardiovascular and metabolic disease domain, validating the pipeline’s effectiveness. 2. The difference between the best model generated by the data-driven subset (AUC 0.891) and the best model generated by the current state of the art hypothesis-driven subset (AUC 0.864) is statistically significant at the 95% confidence interval level. 3. The pipeline itself is highly generic and independent of the specific disease targeted and the EMR used. In conclusion, the application of established machine learning techniques in combination with the proposed pipeline on EMRs has great potential to enhance disease prediction, and hence early detection and intervention in medical practice | |||||||||||||||
Risk of Bias |
|
Paper 28
Paper Name: Natural language processing improves identification of colorectal cancer testing in the electronic medical record
Authors or developers | Denny, J. C. Choma, N. N. Peterson, J. F. Miller, R. A. Bastarache, L. Li, M. Peterson, N. B. | |||||||||||||||
---|---|---|---|---|---|---|---|---|---|---|---|---|---|---|---|---|
Year of Publication | 2012 | |||||||||||||||
Full reference of the study | Denny, J. C., Choma, N. N., Peterson, J. F., Miller, R. A., Bastarache, L., Li, M., & Peterson, N. B. (2012). Natural Language Processing Improves Identification of Colorectal Cancer Testing in the Electronic Medical Record. Medical Decision Making, 32(1), 188�197. https://doi.org/10.1177/0272989X11400418 | |||||||||||||||
Abstract | BACKGROUND: Difficulty identifying patients in need of colorectal cancer (CRC) screening contributes to low screening rates. OBJECTIVE: To use Electronic Health Record (EHR) data to identify patients with prior CRC testing. DESIGN: A clinical natural language processing (NLP) system was modified to identify 4 CRC tests (colonoscopy, flexible sigmoidoscopy, fecal occult blood testing, and double contrast barium enema) within electronic clinical documentation. Text phrases in clinical notes referencing CRC tests were interpreted by the system to determine whether testing was planned or completed and to estimate the date of completed tests. SETTING: Large academic medical center. PATIENTS: 200 patients >= 50 years old who had completed >= 2 non-acute primary care visits within a 1-year period. MEASURES: Recall and precision of the NLP system, billing records, and human chart review were compared to a reference standard of human review of all available information sources. RESULTS: For identification of all CRC tests, recall and precision were as follows: NLP system (recall 93%, precision 94%), chart review (74%, 98%), and billing records review (44%, 83%). Recall and precision for identification of patients in need of screening were: NLP system (recall 95%, precision 88%), chart review (99%, 82%), and billing records (99%, 67%). LIMITATIONS: Small sample size and requirement for a robust EHR. CONCLUSIONS: Applying NLP to EHR records detected more CRC tests than either manual chart review or billing records review alone. NLP had better precision but marginally lower recall to identify patients who were due for CRC screening than billing record review. | |||||||||||||||
Country of Research | USA | |||||||||||||||
Design of Study | Cohort study | |||||||||||||||
Duration of Study | Not Specified | |||||||||||||||
Name of Condition | Colorectal Cancer | |||||||||||||||
Artificial Intelligence Technique Used | Natural language processing (NLP) | |||||||||||||||
Provider’s involvement in | Developing : Unified Medical Language System (UMLS) concepts from biomedical text documents and produces XML-tagged output containing lists of UMLS concepts found in each sentence with relevant context | |||||||||||||||
Accuracy of the AI Intervention | Not specified | |||||||||||||||
Patient-related Outcomes Assessed | Natural language processing NLP system (recall 93%, precision 94%), chart review (74%, 98%), and billing records review (44%, 83%). Recall and precision for identification of patients in need of screening were: NLP system (recall 95%, precision 88%), chart review (99%, 82%), and billing records (99%, 67%). | |||||||||||||||
Primary Healthcare Worker Related Outcomes Assessed | Not specified | |||||||||||||||
Healthcare System-related Outcomes Assessed | Not specified | |||||||||||||||
Reached Target Population? | Yes | |||||||||||||||
Adoption | No | |||||||||||||||
Implementation | Natural language processing NLP system (recall 93%, precision 94%), chart review (74%, 98%), and billing records review (44%, 83%). Recall and precision for identification of patients in need of screening were: NLP system (recall 95%, precision 88%), chart review (99%, 82%), and billing records (99%, 67%). | |||||||||||||||
Maintenance | No | |||||||||||||||
Key Conclusions | Applying Natural language processing NLP to electronic health record EHR detected more colorectal cancer CRC tests than either manual chart review or billing records review alone. NLP had better precision but marginally lower recall to identify patients who were due for CRC screening than billing record review. | |||||||||||||||
Risk of Bias |
|
Paper 29
Paper Name: Defining Disease Phenotypes in Primary Care Electronic Health Records by a Machine Learning Approach: A Case Study in Identifying Rheumatoid Arthritis
Authors or developers | Zhou, S. M. Fernandez-Gutierrez, F. Kennedy, J. Cooksey, R. Atkinson, M. Denaxas, S. Siebert, S. Dixon, W. G.’, “O’Neill, T. W.”, ‘Choy, E. Sudlow, C. U. K. Biobank Follow-up,Outcomes Group,Brophy | |||||||||||||||
---|---|---|---|---|---|---|---|---|---|---|---|---|---|---|---|---|
Year of Publication | 2016 | |||||||||||||||
Full reference of the study | Zhou S-M, Fernandez-Gutierrez F, Kennedy J, Cooksey R, Atkinson M, Denaxas S, et al. (2016) Defining Disease Phenotypes in Primary Care Electronic Health Records by a Machine Learning Approach: A Case Study in Identifying Rheumatoid Arthritis. PLoS ONE 11(5): e0154515. doi:10.1371/journal.pone.0154515 | |||||||||||||||
Abstract | OBJECTIVES: 1) To use data-driven method to examine clinical codes (risk factors) of a medical condition in primary care electronic health records (EHRs) that can accurately predict a diagnosis of the condition in secondary care EHRs. 2) To develop and validate a disease phenotyping algorithm for rheumatoid arthritis using primary care EHRs. METHODS: This study linked routine primary and secondary care EHRs in Wales, UK. A machine learning based scheme was used to identify patients with rheumatoid arthritis from primary care EHRs via the following steps: i) selection of variables by comparing relative frequencies of Read codes in the primary care dataset associated with disease case compared to non-disease control (disease/non-disease based on the secondary care diagnosis); ii) reduction of predictors/associated variables using a Random Forest method, iii) induction of decision rules from decision tree model. The proposed method was then extensively validated on an independent dataset, and compared for performance with two existing deterministic algorithms for RA which had been developed using expert clinical knowledge. RESULTS: Primary care EHRs were available for 2,238,360 patients over the age of 16 and of these 20,667 were also linked in the secondary care rheumatology clinical system. In the linked dataset, 900 predictors (out of a total of 43,100 variables) in the primary care record were discovered more frequently in those with versus those without RA. These variables were reduced to 37 groups of related clinical codes, which were used to develop a decision tree model. The final algorithm identified 8 predictors related to diagnostic codes for RA, medication codes, such as those for disease modifying anti-rheumatic drugs, and absence of alternative diagnoses such as psoriatic arthritis. The proposed data-driven method performed as well as the expert clinical knowledge based methods. CONCLUSION: Data-driven scheme, such as ensemble machine learning methods, has the potential of identifying the most informative predictors in a cost-effective and rapid way to accurately and reliably classify rheumatoid arthritis or other complex medical conditions in primary care EHRs. | |||||||||||||||
Country of Research | UK | |||||||||||||||
Design of Study | Unclear | |||||||||||||||
Duration of Study | ABMU region from March 2009-October 2012 Cardiff from October 2013-July 2014, SAIL databank: 14 years 1999-2013 | |||||||||||||||
Name of Condition | Rheumatoid Arthritis | |||||||||||||||
Artificial Intelligence Technique Used | Random forest method, Algorithm identified 8 predictors related to diagnostic codes for Rheumatoid Arthritis, medication codes, such as those for disease modifying anti-rheumatic drugs, and absence of alternative diagnoses such as psoriatic arthritis. The proposed data-driven method performed as well as the expert clinical knowledge based methods | |||||||||||||||
Providers’ involvement in | Developing : Not specified,Testing : Not specified,Validating : Not specified | |||||||||||||||
Accuracy of the AI Intervention | Overall accuracy of 92.29%, Positive predictive value: 85.6%, specificity: 94.6%, sensitivity: 86.2% | |||||||||||||||
Patient-related Outcomes Assessed | 27% prevalance of rheumatoid arthritis in the assessed population | |||||||||||||||
Primary Healthcare Worker Related Outcomes Assessed | Not specified | |||||||||||||||
Healthcare System-related Outcomes Assessed | Not specified | |||||||||||||||
Reached Target Population? | Not specified | |||||||||||||||
Adoption | No | |||||||||||||||
Implementation | Not implemented but electronic medical records used. | |||||||||||||||
Maintenance | No : The approach was not implemented. | |||||||||||||||
Key Conclusions | The study proposed a data-driven method which performed as well as the expert clinical knowledge based methods to detect rheumatoid arthritis., The findings of this work demonstrate how machine learning methods can be utilized to create reliable disease phenotypes in electronic health records electronic health records. This method may be particularly valuable for large population-based research cohorts to give simple algorithms with good performance that are transparent and easy to apply. This paper has also compared the data-driven methods with the two existing Rheumatoid Arthritis, RA algorithms available for rheumatoid arthritis research using UK datasets, so offers a comparison of performance that can be used by researchers to decide which algorithm is most appropriate to their research. | |||||||||||||||
Risk of Bias |
|
Paper 30
Paper Name: Utilizing uncoded consultation notes from electronic medical records for predictive modeling of colorectal cancer
Authors or developers | Hoogendoorn, M. Szolovits, P. Moons, L. M. G. Numans, M. E. | |||||||||||||||
---|---|---|---|---|---|---|---|---|---|---|---|---|---|---|---|---|
Year of Publication | 2016 | |||||||||||||||
Full reference of the study | Hoogendoorn, Mark, et al. “Utilizing uncoded consultation notes from electronic medical records for predictive modeling of colorectal cancer.” Artificial intelligence in medicine 69 (2016): 53-61. | |||||||||||||||
Abstract | OBJECTIVE: Machine learning techniques can be used to extract predictive models for diseases from electronic medical records (EMRs). However, the nature of EMRs makes it difficult to apply off-the-shelf machine learning techniques while still exploiting the rich content of the EMRs. In this paper, we explore the usage of a range of natural language processing (NLP) techniques to extract valuable predictors from uncoded consultation notes and study whether they can help to improve predictive performance. METHODS: We study a number of existing techniques for the extraction of predictors from the consultation notes, namely a bag of words based approach and topic modeling. In addition, we develop a dedicated technique to match the uncoded consultation notes with a medical ontology. We apply these techniques as an extension to an existing pipeline to extract predictors from EMRs. We evaluate them in the context of predictive modeling for colorectal cancer (CRC), a disease known to be difficult to diagnose before performing an endoscopy. RESULTS: Our results show that we are able to extract useful information from the consultation notes. The predictive performance of the ontology-based extraction method moves significantly beyond the benchmark of age and gender alone (area under the receiver operating characteristic curve (AUC) of 0.870 versus 0.831). We also observe more accurate predictive models by adding features derived from processing the consultation notes compared to solely using coded data (AUC of 0.896 versus 0.882) although the difference is not significant. The extracted features from the notes are shown be equally predictive (i.e. there is no significant difference in performance) compared to the coded data of the consultations. CONCLUSION: It is possible to extract useful predictors from uncoded consultation notes that improve predictive performance. Techniques linking text to concepts in medical ontologies to derive these predictors are shown to perform best for predicting CRC in our EMR dataset. | |||||||||||||||
Country of Research | Netherlands | |||||||||||||||
Design of Study | Unclear | |||||||||||||||
Duration of Study | 4.5 years,Comments : July 1, 2006 and December 31, 2011 | |||||||||||||||
Name of Condition | Colorectal cancer,Comments : consultation notes | |||||||||||||||
Artificial Intelligence Technique Used | Natural language processing extension NLP techniques: a benchmark (bag of words), unsupervised methods to extract information from text (topic modeling), and specifically designed approaches for the case at hand (the remaining approaches). The choices for specific techniques within these categories are based on observations from the literature | |||||||||||||||
Provider’s involvement in | Developing : Not specified,Testing : Not specified,Validating : Not specified | |||||||||||||||
Accuracy of the AI Intervention | AUC: 0.87,Comments : Processing consultation notes: 0.896 | |||||||||||||||
Patient-related Outcomes Assessed | Not specified | |||||||||||||||
Primary Healthcare Worker Related Outcomes Assessed | Not specified | |||||||||||||||
Healthcare System-related Outcomes Assessed | Not specified | |||||||||||||||
Reached Target Population? | Yes | |||||||||||||||
Adoption | Yes (number of providers i.e. PHC participating) : Dutch general practitioners | |||||||||||||||
Implementation | Not specified | |||||||||||||||
Maintenance | No | |||||||||||||||
Key Conclusions | The paper studies several natural language processing (NLP) techniques to extract predictors from uncoded data in electronic medical records (EMRs). Some techniques are well-known while other have been developed specifically for this research. The approaches have been applied to a large dataset we have access to, covering 90,000 patients in general practices. We focus on predictive modelling of colorectal cancer, which is a challenging disease to study as it is a common type of cancer, while the symptoms are very a-specific for the disease. The results show that some of the NLP techniques studied can complement the coded EMR data, and hence, result in improved predictive models. | |||||||||||||||
Risk of Bias |
|